Open Access
ARTICLE
Generating Intelligent Remedial Materials with Genetic Algorithms and Concept Maps
Department of Software Engineering and Management, National Kaohsiung Normal University, Kaohsiung City, 824, Taiwan
* Corresponding Author: Che-Chern Lin. Email:
Intelligent Automation & Soft Computing 2022, 34(2), 1333-1349. https://doi.org/10.32604/iasc.2022.025387
Received 22 November 2021; Accepted 14 January 2022; Issue published 03 May 2022
Abstract
This study proposes an intelligent remedial learning framework to improve students’ learning effectiveness. Basically, this framework combines a genetic algorithm with a concept map in order to select a set of remedial learning units according to students’ weaknesses of learning concepts. In the proposed algorithm, a concept map serves to represent the knowledge structure of learning concepts, and a genetic algorithm performs an iteratively evolutionary procedure in order to establish remedial learning materials based on students’ understanding of these learning concepts. This study also conducted simulations in order to validate the proposed framework using artificially generated data sets, and problematic issues regarding generalizing the special case of the proposed framework are further discussed. The proposed algorithm can be generally-employed in e-learning, providing a framework for generating remedial learning materials for all kinds of learning fields.Keywords
Cite This Article
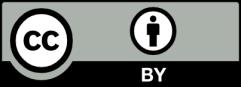
This work is licensed under a Creative Commons Attribution 4.0 International License , which permits unrestricted use, distribution, and reproduction in any medium, provided the original work is properly cited.