Open Access
ARTICLE
Bayesian Convolution for Stochastic Epidemic Model
1 Department of Statistics, Faculty of Mathematics and Natural Sciences, Universitas Halu Oleo, Kendari, 93232, Indonesia
2 Department of Statistics, Faculty of Mathematics and Natural Sciences, Universitas Negeri Makassar, Makassar, 90223, Indonesia
3 Department of Mathematics and Statistics, College of Sciences, Taif University, Taif, 21944, Saudi Arabia
4 Department of Statistics, Mathematics, and Insurance, Faculty of Commerce, Tanta University, Egypt
5 Department of Economics and Finance, College of Business Administration, Taif University, Taif, 21944, Saudi Arabia
6 Department of Mathematics, College of Sciences and Arts, Najran University, Najran, 66445, Saudi Arabia
* Corresponding Author: Mukhsar. Email:
Intelligent Automation & Soft Computing 2022, 34(2), 1175-1186. https://doi.org/10.32604/iasc.2022.025214
Received 16 November 2021; Accepted 14 January 2022; Issue published 03 May 2022
Abstract
Dengue Hemorrhagic Fever (DHF) is a tropical disease that always attacks densely populated urban communities. Some factors, such as environment, climate and mobility, have contributed to the spread of the disease. The Aedes aegypti mosquito is an agent of dengue virus in humans, and by inhibiting its life cycle it can reduce the spread of the dengue disease. Therefore, it is necessary to involve the dynamics of mosquito's life cycle in a model in order to obtain a reliable risk map for intervention. The aim of this study is to develop a stochastic convolution susceptible, infective, recovered-susceptible, infective (SIR-SI) model describing the dynamics of the relationship between humans and Aedes aegypti mosquitoes. This model involves temporal trend and uncertainty factors for both local and global heterogeneity. Bayesian approach was applied for the parameter estimation of the model. It has an intrinsic recurrent logic for Bayesian analysis by including prior distributions. We developed a numerical computation and carry out simulations in WinBUGS, an open-source software package to perform Markov chain Monte Carlo (MCMC) method for Bayesian models, for the complex systems of convolution SIR-SI model. We considered the monthly DHF data of the 2016–2018 periods from 10 districts in Kendari-Indonesia for the application as well as the validation of the developed model. The estimated parameters were updated through to Bayesian MCMC. The parameter estimation process reached convergence (or fulfilled the Markov chain properties) after 50000 burn-in and 10000 iterations. The deviance was obtained at 453.7, which is smaller compared to those in previous models. The districts of Wua-Wua and Kadia were consistent as high-risk areas of DHF. These two districts were considered to have a significant contribution to the fluctuation of DHF cases.Keywords
Cite This Article
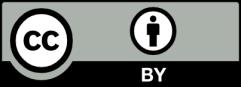
This work is licensed under a Creative Commons Attribution 4.0 International License , which permits unrestricted use, distribution, and reproduction in any medium, provided the original work is properly cited.