Open Access
ARTICLE
Mango Leaf Stress Identification Using Deep Neural Network
1 DIT University, Dehradun, 248003, India
2 Chandigah University, Mohali, 175001, India
* Corresponding Author: Vinay Gautam. Email:
Intelligent Automation & Soft Computing 2022, 34(2), 849-864. https://doi.org/10.32604/iasc.2022.025113
Received 12 November 2021; Accepted 17 January 2022; Issue published 03 May 2022
Abstract
Mango is a widely growing and consumable fruit crop. The quantity and quality of production are most important to satisfy the needs of the huge population. Numerous research has been conducted to increase the yield of the crop. But a good number of crop harvests were destroyed due to various factors and leaf stress is one of them. The various types of stresses include biotic and abiotic that impact the mangoes productivity. But here the focus is on biotic stress factors such as fungus and bacteria. The effect of the stress can be reduced in the preliminary stage by taking some affirmative steps such as earlier detection and resolutions for the same. So many advanced techniques and methods have been used extensively to identify and classify certain stresses. This research supports farmers in identifying early-stage stresses in the plant leaf, that enhances the mango quality and quantity. This approach’s main objective is to ensure that farmers are accurately informed and driven by accurate results of the diagnosis based on affirmative knowledge. The complete approach is laid down in two folds. Firstly, the region of interest is segmented from input images. The region of interest is used to extract symmetrical features of images and these features are used to create symmetry in the classification and identification. Secondly, the segmented image is processed through Convolutional Neural Network (CNN). CNN model is a multilayer model that automatically extracts features from the inputted image. The proposed technique is compared with various models such as VGG16, VGG19, and RestNet, etc. The proposed technique in this paper outperforms other models. The whole experiment was performed using Google Co-Lab using dataset from a known standard or open-source repository. The data collection is composed of different diseases characteristics compared to a healthy leaf. The different stress approach was chosen for different types of mango leaves like powdery mildew, anthracnose, dieback, phoma blight, bacterial canker, and red rust. The result outcomes proved that the proposed approach was 98.12% accurate to identify stress and can be beneficial in actual application by farmers.Keywords
Cite This Article
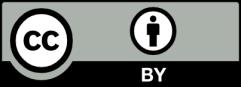