Open Access
ARTICLE
Practical Machine Learning Techniques for COVID-19 Detection Using Chest X-Ray Images
Department of Computer Engineering, King Mongkut’s University of Technology Thonburi, Bangkok, 10140, Thailand
* Corresponding Author: Naruemon Wattanapongsakorn. Email:
Intelligent Automation & Soft Computing 2022, 34(2), 733-752. https://doi.org/10.32604/iasc.2022.025073
Received 10 November 2021; Accepted 07 January 2022; Issue published 03 May 2022
Abstract
This paper presents effective techniques for automatic detection/classification of COVID-19 and other lung diseases using machine learning, including deep learning with convolutional neural networks (CNN) and classical machine learning techniques. We had access to a large number of chest X-ray images to use as input data. The data contains various categories including COVID-19, Pneumonia, Pneumothorax, Atelectasis, and Normal (without disease). In addition, chest X-ray images with many findings (abnormalities and diseases) from the National Institutes of Health (NIH) was also considered. Our deep learning approach used a CNN architecture with VGG16 and VGG19 models which were pre-trained with ImageNet. We compared this approach with the classical machine learning approaches, namely Support Vector Machine (SVM) and Random Forest. In addition to independently extracting image features, pre-trained features obtained from a VGG19 model were utilized with these classical machine learning techniques. Both binary and categorical (multi-class) classification tasks were considered on classical machine learning and deep learning. Several X-ray images ranging from 7000 images up to 11500 images were used in each of our experiments. Five experimental cases were considered for each classification model. Results obtained from all techniques were evaluated with confusion matrices, accuracy, precision, recall and F1-score. In summary, most of the results are very impressive. Our deep learning approach produced up to 97.5% accuracy and 98% F1-score on COVID-19 vs. non-COVID-19 (normal or diseases excluding COVID-19) class, while in classical machine learning approaches, the SVM with pre-trained features produced 98.9% accuracy, and at least 98.2% precision, recall and F1-score on COVID-19 vs. non-COVID-19 class. These disease detection models can be deployed for practical usage in the near future.Keywords
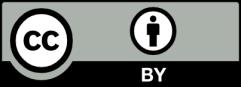