Open Access
ARTICLE
Hybrid Optimized Learning for Lung Cancer Classification
1 Agni College of Technology, Chennai, 600130, India
2 SSN College of Engineering, Chennai, 600004, India
* Corresponding Author: R. Vidhya. Email:
Intelligent Automation & Soft Computing 2022, 34(2), 911-925. https://doi.org/10.32604/iasc.2022.025060
Received 10 November 2021; Accepted 14 January 2022; Issue published 03 May 2022
Abstract
Computer tomography (CT) scan images can provide more helpful diagnosis information regarding the lung cancers. Many machine learning and deep learning algorithms are formulated using CT input scan images for the improvisation in diagnosis and treatment process. But, designing an accurate and intelligent system still remains in darker side of the research side. This paper proposes the novel classification model which works on the principle of fused features and optimized learning network. The proposed framework incorporates the principle of saliency maps as a first tier segmentation, which is then fused with deep convolutional neural networks to improve the classification maps and eventually reduce the risk of overfitting problems. Furthermore, the proposed work has replaced the traditional neural network with Ant-Lion Optimized Feed Forward Layers (ALO_FFL) to obtain the best classification of cancers in Lung CT scan images. The proposed algorithm has been implemented using Tensorflow 1.8 and Keras API with Python 3.8 programming. The extensive experimentations are carried out using the LIDC-IDRI image datasets and various performance metrics such as accuracy, sensitivity, specificity, precision and f1-score are calculated and analyzed. Simulation results show the proposed framework shows 99.89% accuracy, 99.8% sensitivity, 99.76% specificity, 99.8% precision and even 99.88% F1-score respectively. At last, comparative analysis is done with other existing models to prove the excellence of the proposed framework.Keywords
Cite This Article
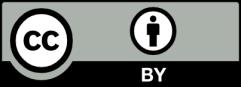
This work is licensed under a Creative Commons Attribution 4.0 International License , which permits unrestricted use, distribution, and reproduction in any medium, provided the original work is properly cited.