Open Access
ARTICLE
Crack Detection in Composite Materials Using McrowDNN
1 Department of CSE, Coimbatore Institute of Technology, Coimbatore, India
2 Department of ECE, Coimbatore Institute of Technology, Coimbatore, India
* Corresponding Author: R. Saveeth. Email:
Intelligent Automation & Soft Computing 2022, 34(2), 983-1000. https://doi.org/10.32604/iasc.2022.023455
Received 09 September 2021; Accepted 16 December 2021; Issue published 03 May 2022
Abstract
In the aerospace industry, composite materials are becoming more common. The presence of a crack in an aircraft makes it weaker and more dangerous, and it can lead to complete fracture and catastrophic failure. To predict the position and depth of a crack, various methods have been developed. For aircraft repair, crack diagnosis is extremely important. Even then, due to uncertainties arising from sources such as environmental conditions, packing, and intrinsic material property changes, accurate diagnosis in real engineering applications remains a challenge. Deep learning (DL) approaches have demonstrated powerful recognition potential in a variety of fields in recent years. In comparison to conventional artificial neural networks, which have the ability to perform better crack recognition, the Deep Neural Network (DNN) is able to improve the pattern features and achieve better pattern recognition. In this study, DNN-based crack detection in composite material method called Modified Crow Deep Neural Network (McrowDNN) is proposed. The preparation of a Mcrow-based DNN is carried out here in order to choose the most appropriate weights and prejudices. The results show that the proposed method outperforms current methods like Artificial Neural Network (ANN), Recurrent Neural Network (RNN) and Crow Search Algorithm (CSA).Keywords
Cite This Article
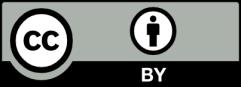
This work is licensed under a Creative Commons Attribution 4.0 International License , which permits unrestricted use, distribution, and reproduction in any medium, provided the original work is properly cited.