Open Access
ARTICLE
Multi-Classification and Distributed Reinforcement Learning-Based Inspection Swarm Offloading Strategy
1 Chengdu University of Information and Technology, Chengdu, 610255, China
2 Southwest Minzu University, Chengdu, 610041, China
3 RMIT University, Melbourne, 3058, Australia
* Corresponding Author: Xi Chen. Email:
Intelligent Automation & Soft Computing 2022, 34(2), 1157-1174. https://doi.org/10.32604/iasc.2022.022606
Received 12 August 2021; Accepted 08 November 2021; Issue published 03 May 2022
Abstract
In meteorological and electric power Internet of Things scenarios, in order to extend the service life of relevant facilities and reduce the cost of emergency repair, the intelligent inspection swarm is introduced to cooperate with monitoring tasks, which collect and process the current scene data through a variety of sensors and cameras, and complete tasks such as emergency handling and fault inspection. Due to the limitation of computing resources and battery life of patrol inspection equipment, it will cause problems such as slow response in emergency and long time for fault location. Mobile Edge Computing is a promising technology, which can improve the quality of service of the swarm by offloading the computing task of the inspection equipment to the edge server nearby the network. In this paper, we study the problem of computing offloading of multi-devices multi-tasks and multi-servers in the intelligent patrol inspection swarm under the condition of a dynamic network environment and limited resources of servers and inspection equipment. An effective adaptive learning offloading strategy based on distributed reinforcement learning and multi-classification is proposed to reduce the task processing delay and energy consumption of the intelligent inspection swarm and improve the service quality. Numerical experimental results demonstrate that the proposed strategy is superior to other offloading strategies in terms of time delay, energy consumption and quality of service.Keywords
Cite This Article
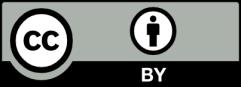
This work is licensed under a Creative Commons Attribution 4.0 International License , which permits unrestricted use, distribution, and reproduction in any medium, provided the original work is properly cited.