Open Access
ARTICLE
Deep Neural Network Based Vehicle Detection and Classification of Aerial Images
1 Department of Computer Science and Engineering, Koneru Lakshmaiah Education Foundation, Vaddeswaram, AP, India
2 Faculty of Engineering & Computer Sciences, Teerthanker Mahaveer University, Moradabad, Uttar Pradesh, India
3 Department of Computer Science and Engineering, Neil Gogte Institute of Technology, Hyderabad, India
4 Department of Computer Science, Faculty of Computers and Information, South Valley University, Qena, 83523, Egypt
5 College of Industrial Engineering, King Khalid University, Abha, Saudi Arabia
6 Department of Information Technology, College of Computers and Information Technology, Taif University, P.O. Box 11099, Taif 21944, Saudi Arabia
* Corresponding Author: Arpit Jain. Email:
Intelligent Automation & Soft Computing 2022, 34(1), 119-131. https://doi.org/10.32604/iasc.2022.024812
Received 01 November 2021; Accepted 22 December 2021; Issue published 15 April 2022
Abstract
The detection of the objects in the ariel image has a significant impact on the field of parking space management, traffic management activities and surveillance systems. Traditional vehicle detection algorithms have some limitations as these algorithms are not working with the complex background and with the small size of object in bigger scenes. It is observed that researchers are facing numerous problems in vehicle detection and classification, i.e., complicated background, the vehicle’s modest size, other objects with similar visual appearances are not correctly addressed. A robust algorithm for vehicle detection and classification has been proposed to overcome the limitation of existing techniques in this research work. We propose an algorithm based on Convolutional Neural Network (CNN) to detect the vehicle and classify it into light and heavy vehicles. The performance of this approach was evaluated using a variety of benchmark datasets, including VEDAI, VIVID, UC Merced Land Use, and the Self database. To validate the results, various performance parameters such as accuracy, precision, recall, error, and F1-Score were calculated. The results suggest that the proposed technique has a higher detection rate, which is approximately 92.06% on the VEDAI dataset, 95.73% on the VIVID dataset, 90.17% on the UC Merced Land dataset, and 96.16% on the Self dataset.Keywords
Cite This Article
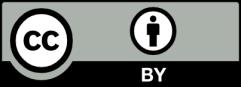
This work is licensed under a Creative Commons Attribution 4.0 International License , which permits unrestricted use, distribution, and reproduction in any medium, provided the original work is properly cited.