Open Access
ARTICLE
Modeling of Chaotic Political Optimizer for Crop Yield Prediction
1 Department of Computer Science and Engineering, Sree Vidyanikethan Engineering College, Tirupati, 517102, India
2 Department of Information Science and Engineering, M S Ramaiah Institute of Technology, Bangalore, 560054, India
3 Department of Computer Science and Engineering, M S Ramaiah Institute of Technology, Bengaluru, 560054, India
4 Department of Computer Science and Engineering, R.V.R & J.C College of Engineering, Guntur Andhra Pradesh, 522529, India
5 Department of Electrical Engineering, Model Institute of Engineering and Technology, J&K, 181122, India
6 Department of CSE, School of Engineering, Bennett University, Greater Noida, 201310, India
7 Faculty of Engineering & Technology, Chhatrapati Shivaji Maharaj University, Navi Mumbai, 410206, India
* Corresponding Author: Gurram Sunitha. Email:
Intelligent Automation & Soft Computing 2022, 34(1), 423-437. https://doi.org/10.32604/iasc.2022.024757
Received 30 October 2021; Accepted 28 December 2021; Issue published 15 April 2022
Abstract
Crop yield is an extremely difficult trait identified using many factors like genotype, environment and their interaction. Accurate Crop Yield Prediction (CYP) necessitates the basic understanding of the functional relativity among yields and the collaborative factor. Disclosing such connection requires both wide-ranging datasets and an efficient model. The CYP is important to accomplish irrigation scheduling and assessing labor necessities for reaping and storing. Predicting yield using various kinds of irrigation is effective for optimizing resources, but CYP is a difficult process owing to the existence of distinct factors. Recently, Deep Learning (DL) approaches offer solutions to complicated data like weather parameters, maturity groups, genotype, etc. In this aspect, this paper presents an Automated Crop Yield Prediction utilizing Chaotic Political Optimizer with Deep Learning (ACYP-CPODL) model. The proposed ACYP-CPODL technique involves different processes namely pre-processing, prediction and parameter optimization. In addition, the hybrid Convolutional Neural Network (CNN) Long-Short Term Memory (LSTM) technique is designed for the prediction process. Moreover, the hyperparameter tuning of the CNN-LSTM approach is performed by the CPO algorithm. The proposed ACYP-CPODL technique has produced an effective result with an MSE of 0.031 and R2 Score of 0.936, whereas the BLSTM model has produced a near-optimal results. As a result, the proposed ACYP-CPODL method has proven to be an effective tool for predicting the crop yields. For validating the improved predictive performance of the ACYP-CPODL technique, a wide range of simulations take place on benchmark datasets and the comparative results highlighted the betterment of the ACYP-CPODL technique over the recent methods.Keywords
Cite This Article
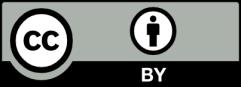
This work is licensed under a Creative Commons Attribution 4.0 International License , which permits unrestricted use, distribution, and reproduction in any medium, provided the original work is properly cited.