Open Access
ARTICLE
Bat-Inspired Optimization for Intrusion Detection Using an Ensemble Forecasting Method
1 Department of Information Technology, E.G.S. Pillay Engineering College, Nagapattinam, Tamilnadu, India
2 Department of Computer Science and Engineering, E.G.S. Pillay Engineering College, Nagapattinam, Tamilnadu, India
* Corresponding Author: R. Anand Babu. Email:
Intelligent Automation & Soft Computing 2022, 34(1), 307-323. https://doi.org/10.32604/iasc.2022.024098
Received 04 October 2021; Accepted 05 November 2021; Issue published 15 April 2022
Abstract
An Intrusion detection system (IDS) is extensively used to identify cyber-attacks preferably in real-time and to achieve integrity, confidentiality, and availability of sensitive information. In this work, we develop a novel IDS using machine learning techniques to increase the performance of the attack detection process. In order to cope with high dimensional feature-rich traffic in large networks, we introduce a Bat-Inspired Optimization and Correlation-based Feature Selection (BIOCFS) algorithm and an ensemble classification approach. The BIOCFS is introduced to estimate the correlation of the identified features and to choose the ideal subset for training and testing phases. The Ensemble Classifier (EC) is used to integrate decisions from three different classifiers including Forest by Penalizing Attributes (FPA), Random Forest (RF), and C4.5 based on the rule of average probabilities. The integration of BIOCFS and EC approaches aids to handle multi-class and unbalanced datasets. The performance of the proposed algorithm is evaluated on a well-known dataset NSL-KDD. The experimental results prove that our combined BIOCFS-EC outdoes other relevant methods in the context of appropriate performance measures. More importantly, the proposed IDS decreases the time complexity of training and testing procedure from 39.43 and 2.25 s to 16.66 and 1.28 s, respectively. Also, the proposed approach achieves the maximum classification accuracy of 0.994, precision of 0.993, F-measure of 0.992, the attack detection ratio of 0.992 and the minimum false alarm ratio of 0.008% on the given dataset.Keywords
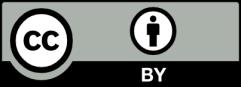