Open Access
ARTICLE
Improve Representation for Cross-Language Clone Detection by Pretrain Using Tree Autoencoder
1 Nanjing University of Aeronautics and Astronautics, Nanjing, 210008, China
2 School of Management Science & Engineering, Nanjing University of Finance and Economics, Nanjing, 210000, China
3 Mcgill University, Montreal, H3G 1Y2, Canada
* Corresponding Author: Dafang Li. Email:
Intelligent Automation & Soft Computing 2022, 33(3), 1561-1577. https://doi.org/10.32604/iasc.2022.027349
Received 15 January 2022; Accepted 20 February 2022; Issue published 24 March 2022
Abstract
With the rise of deep learning in recent years, many code clone detection (CCD) methods use deep learning techniques and achieve promising results, so is cross-language CCD. However, deep learning techniques require a dataset to train the models. The dataset is typically small and has a gap between real-world clones due to the difficulty of collecting datasets for cross-language CCD. This creates a data bottleneck problem: data scale and quality issues will cause that model with a better design can still not reach its full potential. To mitigate this, we propose a tree autoencoder (TAE) architecture. It uses unsupervised learning to pretrain with abstract syntax trees (ASTs) of a large-scale dataset, then fine-tunes the trained encoder in the downstream CCD task. Our proposed TAE contains a tree Long Short-Term Memory (LSTM) encoder and a tree LSTM decoder. We design a novel embedding method for AST nodes, including type embedding and value embedding. In the training of TAE, we present an “encode and decode by layers” strategy and a node-level batch size design. For the CCD dataset, we propose a negative sampling method based on probability distribution. The experimental results on two datasets verify the effeteness of our embedding method, as well as that TAE and its pretrain enhance the performance of the CCD model. The node context information is well captured, and the reconstruction accuracy of the node-value reaches 95.45%. TAE pretrain improves the performance of CCD with a 4% increase in F1 score, which alleviates the data bottleneck problem.Keywords
Cite This Article
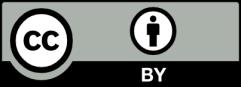