Open Access
ARTICLE
Multi Chunk Learning Based Auto Encoder for Video Anomaly Detection
1 School of Mathematical Sciences, Nanjing Normal University, Nanjing, 210023, China
2 School of Computer and Electronic Information/Artificial Intelligence, Nanjing Normal University, Nanjing, 210023, China
3 Department of Computer Science, University of Massachusetts Boston, Boston, 02125, USA
* Corresponding Author: Genlin Ji. Email:
Intelligent Automation & Soft Computing 2022, 33(3), 1861-1875. https://doi.org/10.32604/iasc.2022.027182
Received 12 January 2022; Accepted 24 February 2022; Issue published 24 March 2022
Abstract
Video anomaly detection is essential to distinguish abnormal events in large volumes of surveillance video and can benefit many fields such as traffic management, public security and failure detection. However, traditional video anomaly detection methods are unable to accurately detect and locate abnormal events in real scenarios, while existing deep learning methods are likely to omit important information when extracting features. In order to avoid omitting important features and improve the accuracy of abnormal event detection and localization, this paper proposes a novel method called Multi Chunk Learning based Skip Connected Convolutional Auto Encoder (MCSCAE). The proposed method improves the accuracy of video anomaly detection by obtaining more vital information. In the data sorting phase, non-uniform chunking is proposed to divide the video frame into several chunks of different sizes to avoid obtaining unnecessary information and omitting crucial information. In order to well reflect the abnormal motion of objects in the video, a new feature, the inter frame flow feature, which is obtained by merging inter frame difference and optical flow features, is proposed to extract motion feature. Moreover, in this paper, skip connection in the auto encoder is utilized during the training phase to reduce the reconstruction error between the original frames and the reconstruction frames, so that the reconstruction error can be used to detect abnormal events during testing. Experience on three public datasets verifies the effectiveness and accuracy of our proposed method. Experimental results show that the proposed method can detect and locate abnormal events outperforms other recent methods significantly.Keywords
Cite This Article
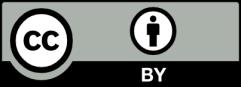