Open Access
ARTICLE
Research on Cross-domain Representation Learning Based on Multi-network Space Fusion
1 School of Artificial Intelligence, Wuxi Vocational College of Science and Technology, Wuxi, 214028, China
2 School of Computer Science and Technology, Harbin Institute of Technology, Weihai, 264209, China
3 Department of Mathematics, Harbin Institute of Technology, Weihai, 264209, China
4 School of Astronautics, Harbin Institute of Technology, Harbin, 150001, China
5 School of Mathematics and Statistics, University College Dublin, Dublin, Ireland
* Corresponding Author: Dongjie Zhu. Email:
Intelligent Automation & Soft Computing 2022, 33(3), 1379-1391. https://doi.org/10.32604/iasc.2022.025181
Received 15 November 2021; Accepted 05 January 2022; Issue published 24 March 2022
Abstract
In recent years, graph representation learning has played a huge role in the fields and research of node clustering, node classification, link prediction, etc., among which many excellent models and methods have emerged. These methods can achieve better results for model training and verification of data in a single space domain. However, in real scenarios, the solution of cross-domain problems of multiple information networks is very practical and important, and the existing methods cannot be applied to cross-domain scenarios, so we research on cross-domain representation is based on multi-network space integration. This paper conducts representation learning research for cross-domain scenarios. First, we use different network representation learning methods to perform representation learning in a single network space. Second, we use the attention mechanism to fuse representations in different spaces to obtain a fusion representation of multiple network spaces; Finally, the model is verified through cross-domain experiments. The experimental results show that the fusion model proposed in this paper can improve the performance of cross-domain scenarios.Keywords
Cite This Article
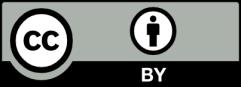
This work is licensed under a Creative Commons Attribution 4.0 International License , which permits unrestricted use, distribution, and reproduction in any medium, provided the original work is properly cited.