Open Access
ARTICLE
Detection of Microbial Activity in Silver Nanoparticles Using Modified Convolution Network
1 Department of Biotechnology, Udaya School of Engineering, Kanyakumari, Tamil Nadu, 629204, India
2 Department of ECE, Ponjesly College of Engineering, Nagercoil, Tamil Nadu, 629003, India
* Corresponding Author: D. Devina Merin. Email:
Intelligent Automation & Soft Computing 2022, 33(3), 1849-1860. https://doi.org/10.32604/iasc.2022.024495
Received 19 October 2021; Accepted 08 December 2021; Issue published 24 March 2022
Abstract
The Deep learning (DL) network is an effective technique that has extended application in medicine, robotics, biotechnology, biometrics and communication. The unique architecture of DL networks can be trained according to classify any complex tasks in a limited duration. In the proposed work a deep convolution neural network of DL is trained to classify the antimicrobial activity of silver nanoparticles (AgNP). The process involves two processing steps; synthesis of silver nanoparticles and classification (SEM) of AgNP based on the antimicrobial activity. AgNP images from scanning electron microscope are pre-processed using Adaptive Histogram Equalization in the networking system and the DL classification model Deep convolution neural network (DCNN) with modified activation function Leaky ReLy is designed particularly to classify the antibacterial activity of AgNP in various concentrations. DCNN analyses the absorption maxima AgNP and categorizes the activity of microbes. The experimental analysis of the proposed method shows that the AgNP shows the maximum absorption value of 0.56 at 450 nm. The overall technique yields an accuracy of 94% through the DCNN technique. The methodology used here to develop silver particles at the nanoscale is easy and economic.Keywords
Cite This Article
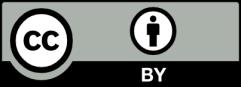
This work is licensed under a Creative Commons Attribution 4.0 International License , which permits unrestricted use, distribution, and reproduction in any medium, provided the original work is properly cited.