Open Access
ARTICLE
MLP-PSO Framework with Dynamic Network Tuning for Traffic Flow Forecasting
1 Department of Computer Science and Engineering, Sri Venkateswara College of Engineering, Sriperumbudur, India
2 Department of Electronics and Communication Engineering, Sri Venkateswara College of Engineering, Sriperumbudur, India
* Corresponding Author: V. Rajalakshmi. Email:
Intelligent Automation & Soft Computing 2022, 33(3), 1335-1348. https://doi.org/10.32604/iasc.2022.024310
Received 13 October 2021; Accepted 13 December 2021; Issue published 24 March 2022
Abstract
Traffic flow forecasting is the need of the hour requirement in Intelligent Transportation Systems (ITS). Various Artificial Intelligence Frameworks and Machine Learning Models are incorporated in today’s ITS to enhance forecasting. Tuning the model parameters play a vital role in designing an efficient model to improve the reliability of forecasting. Hence, the primary objective of this research is to propose a novel hybrid framework to tune the parameters of Multilayer Perceptron (MLP) using the Swarm Intelligence technique called Particle Swarm Optimization (PSO). The proposed MLP-PSO framework is designed to adjust the weights and bias parameters of MLP dynamically using PSO in order to optimize the network performance of MLP. PSO continuously monitors the gradient loss of MLP network while forecasting the traffic flow. The loss is reduced gradually using Inertia Weight (denoted as ω) which is the critical parameter of PSO. It is used to set a balance between the local and global search possibilities. The Inertia Weight has been varied in order to dynamically adjusts the network parameters of MLP. A comparison has been carried out among MLP and MLP-PSO models with variants of Inertia Weight Initializations. The results obtained justifies that, the proposed MLP-PSO framework reduces the forecasting error and improves reliability and accuracy than MLP model.Keywords
Cite This Article
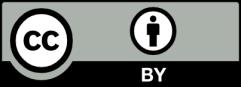
This work is licensed under a Creative Commons Attribution 4.0 International License , which permits unrestricted use, distribution, and reproduction in any medium, provided the original work is properly cited.