Open Access
ARTICLE
Modeling of Hyperparameter Tuned Hybrid CNN and LSTM for Prediction Model
1 Department of Computer Science and Engineering, SRM Institute of Science and Technology, Ramapuram, 600 089, India
2 Department of Computer Science and Engineering, M S Ramaiah Institute of Technology, Bangalore, 560 054, India
3 Department of Information Technology, Sona College of Technology, Salem, 636 005, India
4 Computer Science Department, University of Agriculture Faisalabad, Faisalabad, 38000, Pakistan
5 Department of Computer Science and Engineering, Panimalar Engineering College, Chennai, 600 123, India
6 Department of Computer Science and Engineering, Poornima College of Engineering, Jaipur, 302 022, India
* Corresponding Author: J. Faritha Banu. Email:
Intelligent Automation & Soft Computing 2022, 33(3), 1393-1405. https://doi.org/10.32604/iasc.2022.024176
Received 08 October 2021; Accepted 26 November 2021; Issue published 24 March 2022
Abstract
The stock market is an important domain in which the investors are focused to, therefore accurate prediction of stock market trends remains a hot research area among business-people and researchers. Because of the non-stationary features of the stock market, the stock price prediction is considered a challenging task and is affected by several factors. Anticipating stock market trends is a difficult endeavor that requires a lot of attention, because correctly predicting stock prices can lead to significant rewards if the right judgments are made. Due to non-stationary, noisy, and chaotic data, stock market prediction is a huge difficulty, and as a result, investors find it difficult to invest their money in order to make a profit. In order to predict stock market movements, a number of strategies have been established. Earlier studies based on statistical models and machine learning techniques have been focused on short term stock price prediction. In this aspect, this study designs a novel hyperparameter tuned hybrid convolutional neural network with long short term memory (HPT-HCLSTM) for stock price prediction. The proposed HPT-HCLSTM technique encompasses three different processes namely pre-processing, prediction, and parameter optimization. The HPT-HCLSTM technique employs the HCLSTM technique for the prediction of stock prices. In addition, teaching and learning based optimization (TLBO) algorithm is applied for the hyperparameter optimization of the HCLSTM technique and thereby results in minimal error values. In order to demonstrate the enhanced prediction performance of the HPT-HCLSTM technique, a wide range of simulations were carried out and the results highlighted the better performance of the HPT-HCLSTM technique under several aspects. The HPT-HCLSTM technique is found to be a proper tool for forecasting stock prices. the HPT-HCLSTM technique has showcased better performance with the increased R2 value of 0.9154.Keywords
Cite This Article
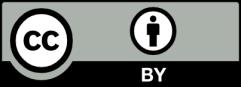
This work is licensed under a Creative Commons Attribution 4.0 International License , which permits unrestricted use, distribution, and reproduction in any medium, provided the original work is properly cited.