Open Access
ARTICLE
Sensor Location Optimisation Design Based on IoT and Geostatistics in Greenhouse
1 Information Center, Jiangsu Academy of Agricultural Sciences, Nanjing, 210014, China
2 Jiangsu Vocational College of Agriculture and Forestry, Jurong, 212400, China
3 Key Laboratory of Agro-Environment in Downstream of Yangtze Plain, Ministry of Agriculture and Rural Affairs/Institute of Agricultural Resources and Environment, Jiangsu Academy of Agricultural Sciences, Nanjing, 210014, China
4 U. S. National Poultry Research Center, Agricultural Research Service, United States Department of Agriculture, Athens, GA 30605, USA
* Corresponding Author: Ni Ren. Email:
Intelligent Automation & Soft Computing 2022, 33(3), 1653-1663. https://doi.org/10.32604/iasc.2022.017049
Received 19 January 2021; Accepted 05 January 2022; Issue published 24 March 2022
Abstract
Environmental parameters such as air temperature (T) and air relative humidity (RH) should be intensively monitored in a greenhouse in real time. In most cases, one set of sensors is installed in the centre of a greenhouse. However, as the microclimate of a greenhouse is always heterogeneous, the sensor installation location is crucial for practical cultivation. In this study, the T and RH monitoring performance of different sensors were compared. Two types of real-time environmental sensors (Air Temperature and Humidity sensor and Activity Monitoring sensor, referred as ATH and AM) were selected and calibrated by reliable non-real-time sensors (Honest Observer By Onset sensor, referred as HOBO). The results showed that T and RH were variable in a small greenhouse area (128 m2). ATH had better T and RH monitoring performance than AM using HOBO as a reference (R2 = 0.968 and 0.938 for T; 0.594 and 0.538 for RH, respectively, for ATH and AM). In terms of cost, it is more efficient to use more sets of AMs (15 sets were used in this case study) to establish an intensive monitoring system based on the Internet of Things (IoT) compared with that of ATH. Then, the optimal sensor installation location was decided using geostatistics. Based on a simulated monitoring data set, the optimal sensor installation location was determined to be 1.57 m away from the physical centre of the monitoring area. By combining IoT with geostatistics, this study offers a method for effective monitoring of environmental parameters in a practical greenhouse system with a three-step procedure.Keywords
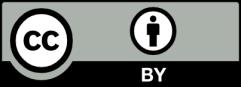