Open Access
ARTICLE
A Novel Convolutional Neural Networks-Fused Shallow Classifier for Breast Cancer Detection
Department of Radiology, College of Medicine, Qassim University, Buraidah, 52571, Saudi Arabia
* Corresponding Author: Sharifa Khalid Alduraibi. Email:
(This article belongs to the Special Issue: Intelligent Systems for Smart and Sustainable Healthcare)
Intelligent Automation & Soft Computing 2022, 33(2), 1321-1334. https://doi.org/10.32604/iasc.2022.025021
Received 08 November 2021; Accepted 13 December 2021; Issue published 08 February 2022
Abstract
This paper proposes a fused methodology based upon convolutional neural networks and a shallow classifier to diagnose and differentiate breast cancer between malignant lesions and benign lesions. First, various pre-trained convolutional neural networks are used to calculate the features of breast ultrasonography (BU) images. Then, the computed features are used to train the different shallow classifiers like the tree, naïve Bayes, support vector machine (SVM), k-nearest neighbors, ensemble, and neural network. After extensive training and testing, the DenseNet-201, MobileNet-v2, and ResNet-101 trained SVM show high accuracy. Furthermore, the best BU features are merged to increase the classification accuracy at the cost of high computational time. Finally, the feature dimension reduction ReliefF algorithm is applied to address the computational complexity issue. An online publicly available dataset of 780 BU images is utilized to validate the proposed approach. The dataset was further divided into 80 and 20 percent ratios for training and testing the models. After extensive testing and comprehensive analysis, it is found that the DenseNet-201 and MobileNet-v2 trained SVM has an accuracy of 90.39% and 94.57% for the original and augmented BU images online dataset, respectively. This study concluded that the proposed framework is efficient and can easily be implemented to help and reduce the workload of radiologists/doctors to diagnose breast cancer in female patients.Keywords
Cite This Article
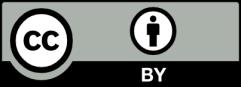