Open Access
ARTICLE
Ensemble Deep Learning Models for Mitigating DDoS Attack in Software-Defined Network
Department of Computer Science, King Abdulaziz University, Jeddah, Saudi Arabia
* Corresponding Author: Fatmah Alanazi. Email:
Intelligent Automation & Soft Computing 2022, 33(2), 923-938. https://doi.org/10.32604/iasc.2022.024668
Received 27 October 2021; Accepted 30 November 2021; Issue published 08 February 2022
Abstract
Software-defined network (SDN) is an enabling technology that meets the demand of dynamic, adaptable, and manageable networking architecture for the future. In contrast to the traditional networks that are based on a distributed control plane, the control plane of SDN is based on a centralized architecture. As a result, SDNs are susceptible to critical cyber attacks that exploit the single point of failure. A distributed denial of service (DDoS) attack is one of the most crucial and risky attacks, targeting the SDN controller and disrupting its services. Several researchers have proposed signature-based DDoS mitigation and detection techniques that rely on manually configuring the policies. As the massive traffic from heterogeneous networks increases, conventional solutions are ineffective due to the lack of automation and human interference. This necessitates producing a detection solution, more effective than traditional ones, to ensure SDN security, resiliency, and availability. This paper addresses this problem by proposing a deep learning (DL)-based ensemble solution for efficient DDoS attack detection in SDN. Four hybrid models are presented by adopting three ensemble techniques and different DL architectures, namely convolutional neural network, long short-term memory, and gated recurrent unit, to improve the SDN traffic classification. The experimentation was conducted on the benchmark flow-based dataset CICIDS2017. High detection accuracy (99.77%) with a small number of flow-based features was achieved by our ensemble model, as our experimental results will demonstrate. The proposed solution was evaluated by several standard assessment matrices and by comparing against other state-of-the-art algorithms from the network security literature.Keywords
Cite This Article
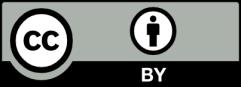
This work is licensed under a Creative Commons Attribution 4.0 International License , which permits unrestricted use, distribution, and reproduction in any medium, provided the original work is properly cited.