Open Access
ARTICLE
Hyperparameter Tuned Bidirectional Gated Recurrent Neural Network for Weather Forecasting
1 Department of Information Technology, Karpagam College of Engineering, Coimbatore, 641032, India
2 Principal Rathiniam Technical Campus, Coimbatore, 641021, India
* Corresponding Author: S. Manikandan. Email:
Intelligent Automation & Soft Computing 2022, 33(2), 761-775. https://doi.org/10.32604/iasc.2022.023398
Received 06 September 2021; Accepted 10 November 2021; Issue published 08 February 2022
Abstract
Weather forecasting is primarily related to the prediction of weather conditions that becomes highly important in diverse applications like drought discovery, severe weather forecast, climate monitoring, agriculture, aviation, telecommunication, etc. Data-driven computer modelling with Artificial Neural Networks (ANN) can be used to solve non-linear problems. Presently, Deep Learning (DL) based weather forecasting models can be designed to accomplish reasonable predictive performance. In this aspect, this study presents a Hyper Parameter Tuned Bidirectional Gated Recurrent Neural Network (HPT-BiGRNN) technique for weather forecasting. The HPT-BiGRNN technique aims to utilize the past weather data for training the BiGRNN model and achieve the effective forecasts with minimum time duration. The BiGRNN is an enhanced version of Gated Recurrent Unit (GRU) that follows the process of passing input via forward and backward neural network and the outputs are linked to the identical output layer. The BiGRNN technique includes several hyper-parameters and hence, the hyperparameter optimization process takes place using Bird Mating Optimizer (BMO). The design of BMO algorithm for hyperparameter optimization of the BiGRNN, particularly for weather forecast shows the novelty of the work. The BMO algorithm is used to set hyperparameters such as momentum, learning rate, batch size and weight decay. The experimental result the HPT-BiGRNN approach has resulted in a lower RMSE of 0.173 whereas the Fuzzy-GP, Fuzzy-SC, MLP-ANN and RBF-ANN methods have gained an increased RMSE of 0.218, 0.216, 0.202 and 0.245 respectively.Keywords
Cite This Article
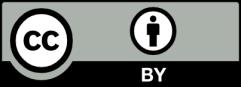
This work is licensed under a Creative Commons Attribution 4.0 International License , which permits unrestricted use, distribution, and reproduction in any medium, provided the original work is properly cited.