Open Access
ARTICLE
Cat-Inspired Deep Convolutional Neural Network for Bone Marrow Cancer Cells Detection
1 Department of ECE, Bharathiyar Institute of Engineering for Women, Deviyakurichi, 636112, India
2 Department of ECE, Mahendra Engineering College, Namakkal, 637503, India
* Corresponding Author: R. Kavitha. Email:
Intelligent Automation & Soft Computing 2022, 33(2), 1305-1320. https://doi.org/10.32604/iasc.2022.022816
Received 19 August 2021; Accepted 06 December 2021; Issue published 08 February 2022
Abstract
Bone marrow cancer is considered to be the most complex and dangerous disease which results due to an uncontrolled growth of white blood cells called leukocytes. Acute Lymphoblastic Leukemia (ALL) and Multiple Myeloma (MM) are considered to be important categories of bone cancers, which induces a larger number of cancer cells in the bone marrow, results in preventing the production of healthy blood cells. The advent of Artificial Intelligence, especially machine and deep learning, has expanded humanity’s capacity to analyze and detect these increasingly complex diseases. But, accurate detection of cancer cells and reducing the probability of false alarm rates remain on the darker side of the research. This paper proposes novel deep convolutional neural networks in which the hyper parameters are optimized by adaptive Multi-objective CAT algorithms. The proposed model is trained on the preprocessed cell images followed by training with Optimized Convolutional Neural Network (OCNN) and finally detecting the category of cancer cells present in the bone marrow. The extensive experimentations have been carried out using SN-AM datasets and various performance metrics such as accuracy, precision, recall, specificity, and F1-score are calculated and analyzed. The overall accuracy was found to be 99.45% in predicting different categories of cancer and it outperforms the pre-trained deep learning models such as Alexnets, VGG-16 nets, and U-nets. To establish the superiority of the proposed model, we have compared the performance of the proposed model with other machine learning models such as Support Vector Machines, Random Forest, Naïve Bayes, and Artificial Neural Networks. From the above intensive studies, it is clear that the proposed model was able to produce brighter performances than the other learning models in detecting the category of cancer cells.Keywords
Cite This Article
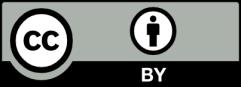
This work is licensed under a Creative Commons Attribution 4.0 International License , which permits unrestricted use, distribution, and reproduction in any medium, provided the original work is properly cited.