Open Access
ARTICLE
Error Calibration Model of Air Pressure Sensor Based on DF-RBF
1 Beijing University of Technology, Beijing, 100124, China
2 Beijing Laboratory of Advanced Information Networks, Beijing, 100124, China
3 Beijing Key Laboratory of Computational Inteligence and Inteligent System, Beijing, 100124, China
4 Department of Computer Science, University of Pittsburgh, Pittsburgh, 15260, USA
5 Qinghai Nationalities University School of Physics and Electronic Information Engineering, Qinghai, 810000, China
* Corresponding Author: Pengyu Liu. Email:
Intelligent Automation & Soft Computing 2022, 33(2), 855-864. https://doi.org/10.32604/iasc.2022.022380
Received 05 August 2021; Accepted 30 September 2021; Issue published 08 February 2022
Abstract
The development of upper-air meteorological detection is contingent upon the improvement of detection instruments. Air pressure sensors play a key role in high altitude meteorological measurement, but they can be frequently affected by temperature fluctutations, resulting in less accurate measurement data. The need to address this limitation has served as the core problem for meteorological detection and drawn great attention from the community. In this paper, we propose a calibration model for the DF-RBF air pressure sensor. The proposed method decomposes the detection process and corrects the measurements by fitting the residuals to true pressure values. In particular, we first calculate the error (i.e., residual) between the measured pressure value and the true pressure values, and then build an analytical formula to represent the relationship between the measurement residual and the temperature. Then we decompose the function and fit its parameters through an RBF network. Finally, we generate the calibrated value by combining the measured value and the residual estimated by the analytical formula. In our experiments, we compare against a baseline method which predicts air pressure directly using temperature by fitting an RBF network. We observe that our proposed method, which combines sensor measurement and residual modeling, can achieve much lower measurement error (reduced from 1.5 to 0.7, over 53% error). This shows the feasibility and potential of the proposed method for barometric calibration.Keywords
Cite This Article
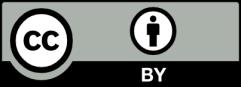