Open Access
ARTICLE
A Machine-Learning Framework to Improve Wi-Fi Based Indoorpositioning
1 CSE, SRC, SASTRA Deemed to Be University, Thanjavur, 613401, India
2 CSE, SOC, SASTRA Deemed to Be University, Thanjavur, 613401, India
* Corresponding Author: K. R. Manjula. Email:
(This article belongs to the Special Issue: Soft Computing Methods for Intelligent Automation Systems)
Intelligent Automation & Soft Computing 2022, 33(1), 383-397. https://doi.org/10.32604/iasc.2022.023105
Received 28 August 2021; Accepted 10 November 2021; Issue published 05 January 2022
Abstract
The indoor positioning system comprises portable wireless devices that aid in finding the location of people or objects within the buildings. Identification of the items is through the capacity level of the signal received from various access points (i.e., Wi-Fi routers). The positioning of the devices utilizing some algorithms has drawn more attention from the researchers. Yet, the designed algorithm still has problems for accurate floor planning. So, the accuracy of position estimation with minimum error is made possible by introducing Gaussian Distributive Feature Embedding based Deep Recurrent Perceptive Neural Learning (GDFE-DRPNL), a novel framework. Novel features from the dataset are through two processing stages dimensionality reduction and position estimation. Initially, the essential elements selection using the Gaussian Distributive Feature Embedding technique is the novel framework. The feature reduction process aims to reduce the time consumption and overhead for estimating the location of various devices. In the next stage, employ Deep Recurrent multilayer Perceptive Neural Learning to evaluate the device position with dimensionality reduced features. The proposed Deep-learning approach accurately learns the quality and the signal strength data with multiple layers by applying Deming Regressive Trilateral Positioning Model. As a result, the GDFE-DRPNL framework increases the positioning accuracy and minimizes the error rate. The experimental assessments with various factors such as positioning accuracy minimized by 70% and 60%, computation time minimized by 45% and 55% as well as overhead by 11% and 23% compared with PFRL and two-dimensional localization algorithm. Through the experiment and after analyzing the data, verify that the proposed GDFE-DRPNL algorithm in this paper is better than the previous methods.Keywords
Cite This Article
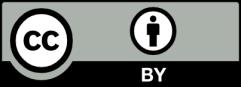
This work is licensed under a Creative Commons Attribution 4.0 International License , which permits unrestricted use, distribution, and reproduction in any medium, provided the original work is properly cited.