Open Access
ARTICLE
Adaptive XGBOOST Hyper Tuned Meta Classifier for Prediction of Churn Customers
1 Department of Computer Science and Engineering, SRK Institute of Technology, Vijayawada, 521108, India
2 Department of Information Technology, PVP Siddhartha Institute of Technology, Vijayawada, 520007, India
3 Department of Computer Science and Engineering, Seshadri Rao Gudlavalleru Engineering College, Gudlavalleru, 521356, India
4 Department of Information Technology, Seshadri Rao Gudlavalleru Engineering College, Gudlavalleru, 521356, India
5 Department of Computer Science and Engineering, SRKR Engineering College, Bhimavaram, 534204, India
6 Department of Computer Science and Engineering, Eswar College of Engineering, Narasaraopeta, 522601, India
* Corresponding Author: B. Srikanth. Email:
Intelligent Automation & Soft Computing 2022, 33(1), 21-34. https://doi.org/10.32604/iasc.2022.022423
Received 07 August 2021; Accepted 29 October 2021; Issue published 05 January 2022
Abstract
In India, the banks have a formidable edge in maintaining their customer retention ratio for past few decades. Downfall makes the private banks to reduce their operations and the nationalised banks merge with other banks. The researchers have used the traditional and ensemble algorithms with relevant feature engineering techniques to better classify the customers. The proposed algorithm uses a Meta classifier instead of an ensemble algorithm with an adaptive genetic algorithm for feature selection. Churn prediction is the number of customers who wants to terminate their services in the banking sector. The model considers twelve attributes like credit score, geography, gender, age, etc, to predict customer churn. The project consists of five modules as follows. First is the pre-processing module that identifies the missing data and fills the value with mean and mode. Second is the data transformation module where, the categorical data is converted into numerical data using label encoding to fasten the computations. The converted numerical data is normalized using the standard scalar technique. The feature selection module identifies the essential attributes using DragonFly and Firefly (Hybrid Fly) algorithms. The classification module designs an intelligent Meta learner, which combines the Ensemble Algorithm Extreme Gradient Boosting (XGBOOST) with base classifiers as “Extra Tree Classifier” and “Logistic Regression” to predict the churn customers.Keywords
Cite This Article
B. Srikanth, S. Lakshmi V. Papineni, G. Sridevi, D. N. V. S. L. S. Indira, K. S. R. Radhika et al., "Adaptive xgboost hyper tuned meta classifier for prediction of churn customers," Intelligent Automation & Soft Computing, vol. 33, no.1, pp. 21–34, 2022. https://doi.org/10.32604/iasc.2022.022423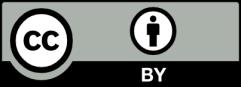