Open Access
ARTICLE
Insider Threat Detection Based on NLP Word Embedding and Machine Learning
1 Department of Computer Science, College of Computer and Information Sciences, Majmaah University, Al-Majmaah 11952, Saudi Arabia
2 Department of Information Technology, College of Computer and Information Sciences, Majmaah University, Al-Majmaah 11952, Saudi Arabia
* Corresponding Author: Mohd Abdul Rahim Khan. Email:
(This article belongs to the Special Issue: Humans and Cyber Security Behaviour)
Intelligent Automation & Soft Computing 2022, 33(1), 619-635. https://doi.org/10.32604/iasc.2022.021430
Received 02 July 2021; Accepted 09 November 2021; Issue published 05 January 2022
Abstract
The growth of edge computing, the Internet of Things (IoT), and cloud computing have been accompanied by new security issues evolving in the information security infrastructure. Recent studies suggest that the cost of insider attacks is higher than the external threats, making it an essential aspect of information security for organizations. Efficient insider threat detection requires state-of-the-art Artificial Intelligence models and utility. Although significant have been made to detect insider threats for more than a decade, there are many limitations, including a lack of real data, low accuracy, and a relatively low false alarm, which are major concerns needing further investigation. In this paper, an attempt to fulfill these gaps by detecting insider threats with the novelties of the present investigation first developed two deep learning hybrid LSTM models integrated with Google's Word2vec LSTM (Long Short-Term Memory) GLoVe (Global Vectors for Word Representation) LSTM. Secondly, the performance of two hybrid DL models was compared with the state-of-the-art ML models such as XGBoost, AdaBoost, RF (Random Forest), KNN (K-Nearest Neighbor) and LR (Logistics Regression). Thirdly, the present investigation bridges the gaps of using a real dataset, high accuracy, and significantly lower false alarm rate. It was found that ML-based models outperformed the DL-based ones. The results were evaluated based on earlier studies and deemed efficient at detecting insider threats using the real dataset.Keywords
Cite This Article
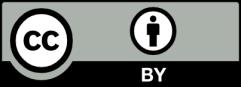
This work is licensed under a Creative Commons Attribution 4.0 International License , which permits unrestricted use, distribution, and reproduction in any medium, provided the original work is properly cited.