Open Access
ARTICLE
Bidirectional Long Short-Term Memory Network for Taxonomic Classification
1 Department of Information Technology, College of Computer and Information Sciences, Princess Nourah Bint Abdulrahman University, Riyadh, Saudi Arabia
2 Department of Electronics and Electrical Communications Engineering, Faculty of Electronic Engineering, Menoufia University, Menoufia, 32952, Egypt
3 Department of Computer Science and Engineering, Faculty of Electronic Engineering, Menoufia University, Menoufia, 32952, Egypt
4 Ministry of Education, Riyadh, Saudi Arabia
* Corresponding Author: Naglaa. F. Soliman. Email:
Intelligent Automation & Soft Computing 2022, 33(1), 103-116. https://doi.org/10.32604/iasc.2022.017691
Received 07 February 2021; Accepted 23 September 2021; Issue published 05 January 2022
Abstract
Identifying and classifying Deoxyribonucleic Acid (DNA) sequences and their functions have been considered as the main challenges in bioinformatics. Advances in machine learning and Deep Learning (DL) techniques are expected to improve DNA sequence classification. Since the DNA sequence classification depends on analyzing textual data, Bidirectional Long Short-Term Memory (BLSTM) algorithms are suitable for tackling this task. Generally, classifiers depend on the patterns to be processed and the pre-processing method. This paper is concerned with a new proposed classification framework based on Frequency Chaos Game Representation (FCGR) followed by Discrete Wavelet Transform (DWT) and BLSTM. Firstly, DNA strings are transformed into numerical matrices by FCGR. Then, the DWT is used instead of the pooling layer as a tool of data compression. The benefit of using the DWT is two-fold. It preserves the useful information only that enables the following BLSTM training, effectively. Besides, DWT adds more important details to the encoded sequences due to finding effective features in the DNA fragments. Finally, the BLSTM model is trained to classify the DNA sequences. Evaluation metrics such as F1 score and accuracy show that the proposed framework outperforms the state-of-the-art algorithms. Hence, it can be used in DNA classification applications.Keywords
Cite This Article
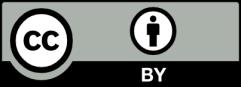