Open Access
ARTICLE
Improved U-Net-Based Novel Segmentation Algorithm for Underwater Mineral Image
1 School of Information and Engineering, Minzu University of China, Beijing, 100081, China
2 Key Laboratory of Marine Environmental Survey Technology and Application, Ministry of Natural Resource, Guangzhou, 510300, China
3 National Language Resource Monitoring & Research Center of Minority Languages, Beijing, 100081, China
4 School of Ocean Science, China University of Geosciences, Beijing, 100191, China
5 Department of Buoy Engineering, South China Sea Marine Survey and Technology Center of State Oceanic Administration, Guangzhou, 510300, China
* Corresponding Author: Wei Song. Email:
Intelligent Automation & Soft Computing 2022, 32(3), 1573-1586. https://doi.org/10.32604/iasc.2022.023994
Received 29 September 2021; Accepted 08 November 2021; Issue published 09 December 2021
Abstract
Autonomous underwater vehicle (AUV) has many intelligent optical system, which can collect underwater signal information to make the system decision. One of them is the intelligent vision system, and it can capture the images to analyze. The performance of the particle image segmentation plays an important role in the monitoring of underwater mineral resources. In order to improve the underwater mineral image segmentation performance, some novel segmentation algorithm architectures are proposed. In this paper, an improved mineral image segmentation is proposed based on the modified U-Net. The pyramid upsampling module and residual module are bring into the U-Net model, which are called JPU-Net, JPMU-Net and ResU-Net. These models combined the power of the residual block and the pyramid upsampling in the encoder part and in the decoder part respectively. The proposed models are tested on the Electron Microscopy images (EM) dataset and the underwater mineral image dataset. The experimental results show that JPU-Net has superior performance on the EM dataset, and JPMU-Net has a better segmentation result than existing convolutional neural network on the underwater mineral image dataset.Keywords
Cite This Article
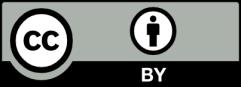
This work is licensed under a Creative Commons Attribution 4.0 International License , which permits unrestricted use, distribution, and reproduction in any medium, provided the original work is properly cited.