Open Access
ARTICLE
Covid-19 Symptoms Periods Detection Using Transfer-Learning Techniques
1 Computer Sciences Program, Turabah University College, Taif University, P. O. Box 11099, Taif 21944, Saudi Arabia
2 College of Applied Computer Sciences, King Saud University, Riyadh, P. O. Box 11451, Saudi Arabia
3 Artificial Intelligence Center of Advanced Studies (Thakaa), King Saud University, Riyadh, P. O. Box 145111, Saudi Arabia
4 Department of Computer Engineering, College of Computer and Information Sciences, King Saud University, Riyadh, 11543, Saudi Arabia
5 Department of Information Technology, College of Computer and Information Science, King Saud University, Riyadh, 11543, Saudi Arabia
* Corresponding Author: Mohammed Faisal. Email:
Intelligent Automation & Soft Computing 2022, 32(3), 1921-1937. https://doi.org/10.32604/iasc.2022.022559
Received 11 August 2021; Accepted 25 October 2021; Issue published 09 December 2021
Abstract
The inflationary illness caused by extreme acute respiratory syndrome coronavirus in 2019 (COVID-19) is an infectious and deadly disease. COVID-19 was first found in Wuhan, China, in December 2019, and has since spread worldwide. Globally, there have been more than 198 M cases and over 4.22 M deaths, as of the first of Augest, 2021. Therefore, an automated and fast diagnosis system needs to be introduced as a simple, alternative diagnosis choice to avoid the spread of COVID-19. The main contributions of this research are 1) the COVID-19 Period Detection System (CPDS), that used to detect the symptoms periods or classes, i.e., healthy period, which mean the no COVID19, the period of the first six days of symptoms (i.e., COVID-19 positive cases from day 1 to day 6), and the third period of infection more than six days of symptoms (i.e., COVID-19 positive cases from day 6 and more): 2) the COVID19 Detection System (CDS) that used to determine if the X-ray images normal, i.e., healthy case or infected, i.e., COVID-19 positive cases; 3) the collection of database consists of three different categories or groups based on the basis of time interval of offset of Symptoms. For CPDS, the VGG-19 perform to 96% accuracy, 90% F1 score, 91% average precision, and 91% average recall. For CDS, the VGG-19 perform to 100% accuracy, 99% F1 score, 100% average precision, and 99% average recall.Keywords
Cite This Article
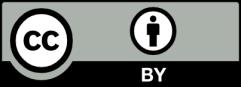
This work is licensed under a Creative Commons Attribution 4.0 International License , which permits unrestricted use, distribution, and reproduction in any medium, provided the original work is properly cited.