Open Access
ARTICLE
Hybrid Deep Learning Framework for Privacy Preservation in Geo-Distributed Data Centre
1 Department of Computer Science and Engineering, Bannari Amman Institute of Technology, Sathyamangalam, 638401, India
2 Department of Information Technology, K.S.R. College of Engineering (Autonomous), Tiruchengode, 637215, India
* Corresponding Author: S. Nithyanantham. Email:
Intelligent Automation & Soft Computing 2022, 32(3), 1905-1919. https://doi.org/10.32604/iasc.2022.022499
Received 10 August 2021; Accepted 18 September 2021; Issue published 09 December 2021
Abstract
In recent times, a huge amount of data is being created from different sources and the size of the data generated on the Internet has already surpassed two Exabytes. Big Data processing and analysis can be employed in many disciplines which can aid the decision-making process with privacy preservation of users’ private data. To store large quantity of data, Geo-Distributed Data Centres (GDDC) are developed. In recent times, several applications comprising data analytics and machine learning have been designed for GDDC. In this view, this paper presents a hybrid deep learning framework for privacy preservation in distributed DCs. The proposed model uses Deep Neural Network (DNN) for the feature extractor and classifier operations. In addition, Siamese training method is applied to fine-tune the prevention of secondary inference on the data. Moreover, gradient descent approach is employed to reduce the loss function of the DNN model. Furthermore, Glow-worm Swarm Optimization (GSO) algorithm is utilized to fine tune the hyperparameters of the DNN model to improve the overall efficiency. The proposed model is executed on a Hadoop based environment, i.e., Hadoop Distributed File System (HDFS), which has two nodes namely master node and slave nodes. The master node is considered as the main user node to get the services from the service provider. Every slave node behaves as per master node’s instruction for data storage. In order to validate the enhanced performance of the proposed model, a series of simulations take place and the experimental results demonstrate the promising performance of the proposed model. The simple technique has reached a maximum gender recognition accuracy of 95, 90 and 79 on the applied data 1, 2 and 3 respectively. Also, the reduced simple approach has attained reduced gender recognition with the accuracy of 91, 84 and 74 on the applied data 1, 2 and 3 respectively.Keywords
Cite This Article
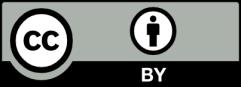
This work is licensed under a Creative Commons Attribution 4.0 International License , which permits unrestricted use, distribution, and reproduction in any medium, provided the original work is properly cited.