Open Access
ARTICLE
Handling High Dimensionality in Ensemble Learning for Arrhythmia Prediction
Department of Computer Science, King Khalid University, Abha, Kingdom of Saudi Arabia
* Corresponding Author: Fuad Ali Mohammed Al-Yarimi. Email:
Intelligent Automation & Soft Computing 2022, 32(3), 1729-1742. https://doi.org/10.32604/iasc.2022.022418
Received 06 August 2021; Accepted 20 October 2021; Issue published 09 December 2021
Abstract
Computer-aided arrhythmia prediction from ECG (electrocardiograms) is essential in clinical practices, which promises to reduce the mortality caused by inexperienced clinical practitioners. Moreover, computer-aided methods often succeed in the early detection of arrhythmia scope from electrocardiogram reports. Machine learning is the buzz of computer-aided clinical practices. Particularly, computer-aided arrhythmia prediction methods highly adopted machine learning methods. However, the high dimensionality in feature values considered for the machine learning models’ training phase often causes false alarming. This manuscript addressed the high dimensionality in the learning phase and proposed an (Ensemble Learning method for Arrhythmia Prediction) ELAP (ensemble learning-based arrhythmia prediction). The proposed method is working as a classification approach that incorporates both supervised and unsupervised learning methods. The experimental study addresses the rise of the proposed method in the prediction accuracy of both labels. The cross-validation statistics of ELAP have been compared to contemporary methods to scale the performance of the ELAP. The proposed method ELAP. Concerning scale, the prediction accuracy, the scope of the false alarm, the robustness of the label prediction, the outcomes of the assessment metrics obtained from 10-fold leave pair out cross-validation performed on proposed ELAP has compared to the corresponding outcomes metrics obtained from the contemporary methods.Keywords
Cite This Article
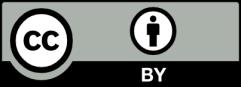
This work is licensed under a Creative Commons Attribution 4.0 International License , which permits unrestricted use, distribution, and reproduction in any medium, provided the original work is properly cited.