Open Access
ARTICLE
An Automated Word Embedding with Parameter Tuned Model for Web Crawling
1 Department of Information Technology, Jeppiaar Institute of Technology, Sriperumbudur, 631604, India
2 Department of Computer Science and Engineering, SRM Institute of Science and Technology, Kattankulathur, 603203, India
3 Department of Computer Science and Engineering, Kakatiya Institute of Technology & Science, Warangal, 506 015, India
4 Department of Electronics Engineering, Shri Ramdeobaba College of Engineering and Management, Nagpur, 440013 India
5 Department of Mechanical Engineering, Vignan's Foundation for Science Technology and Research, Guntur, 522213, India
6 Department of Computer Science and Engineering, Rajalakshmi Institute of Technology, Chennai, 600 124, India
* Corresponding Author: S. Neelakandan. Email:
Intelligent Automation & Soft Computing 2022, 32(3), 1617-1632. https://doi.org/10.32604/iasc.2022.022209
Received 31 July 2021; Accepted 04 October 2021; Issue published 09 December 2021
Abstract
In recent years, web crawling has gained a significant attention due to the drastic advancements in the World Wide Web. Web Search Engines have the issue of retrieving massive quantity of web documents. One among the web crawlers is the focused crawler, that intends to selectively gather web pages from the Internet. But the efficiency of the focused crawling can easily be affected by the environment of web pages. In this view, this paper presents an Automated Word Embedding with Parameter Tuned Deep Learning (AWE-PTDL) model for focused web crawling. The proposed model involves different processes namely pre-processing, Incremental Skip-gram Model with Negative Sampling (ISGNS) based word embedding, bidirectional long short-term memory-based classification and bird swarm optimization based hyperparameter tuning. The SGNS training desires to go over the complete training data to pre-compute the noise distribution before performing Stochastic Gradient Descent (SGD) and the ISGNS technique is derived for the word embedding process. Besides, the cosine similarity is computed from the word embedding matrix to generate a feature vector which is fed as input into the Bidirectional Long Short-Term Memory (BiLSTM) for the prediction of website relevance. Finally, the Birds Swarm Optimization-Bidirectional Long Short-Term Memory (BSO-BiLSTM) based classification model is used to classify the webpages and the BSO algorithm is employed to determine the hyperparameters of the BiLSTM model so that the overall crawling performance can be considerably enhanced. For validating the enhanced outcome of the presented model, a comprehensive set of simulations are carried out and the results are examined in terms of different measures. The Automated Word Embedding with Parameter Tuned Deep Learning (AWE-PTDL) technique has attained a higher harvest rate of 85% when compared with the other techniques. The experimental results highlight the enhanced web crawling performance of the proposed model over the recent state of art web crawlers.Keywords
Cite This Article
Citations
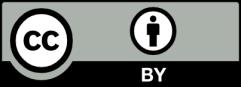
This work is licensed under a Creative Commons Attribution 4.0 International License , which permits unrestricted use, distribution, and reproduction in any medium, provided the original work is properly cited.