Open Access
REVIEW
Comparative Research Directions of Population Initialization Techniques using PSO Algorithm
1 Department of Computer Science, University of Gujrat, Gujrat, 50700, Pakistan
2School of Computer Science, Beijing Institute of Technology, Beijing, 100081, China
3 Govt. College Women University Sialkot, Sialkot, 51310, Pakistan
4 Faculty of Computing and Informatics, University Malaysia Sabah, Jalan UMS, 88400, KK, Malaysia
5 Faculty of Computing & Informatics, Multimedia University, Persiaran Multimedia, Cyberjaya, 63100, Selangor, Malaysia
6 NCRA-Condition Monitoring Systems Lab, Mehran University of Engineering & Technology, Jamshoro, Pakistan
7 Department of Neurobiology and Behavior, University of California Irvine, Irvine, CA, USA
8 Federal University of Piauí (UFPI), Teresina, PI, Brazil
9 Instituto de Telecomunicações, Covilhã, 6201-001, Portugal
10 Department of Electrical Engineering and Computer Science, Howard University, Washington, DC, USA
* Corresponding Author: Kashif Nisar. Email:
Intelligent Automation & Soft Computing 2022, 32(3), 1427-1444. https://doi.org/10.32604/iasc.2022.017304
Received 26 January 2021; Accepted 17 April 2021; Issue published 09 December 2021
Abstract
In existing meta-heuristic algorithms, population initialization forms a huge part towards problem optimization. These calculations can impact variety and combination to locate a productive ideal arrangement. Especially, for perceiving the significance of variety and intermingling, different specialists have attempted to improve the presentation of meta-heuristic algorithms. Particle Swarm Optimization (PSO) algorithm is a populace-based, shrewd stochastic inquiry strategy that is motivated by the inherent honey bee swarm food search mechanism. Population initialization is an indispensable factor in the PSO algorithm. To improve the variety and combination factors, rather than applying the irregular circulation for the introduction of the populace, semi-arbitrary successions are more helpful. This examination presents a thorough overview of the different PSO initialization approaches which are dependent on semi-arbitrary successions systems. In this precise review, the best in class in the populace instatement is uncovered. The procedures are classified by utilizing a theoretical model that parts the cycle of populace introduction into two phases: that is, right now expressly or certainly utilized for reinstatement in every single present approach. The deliberate investigation unveils the potential examination zones of populace introduction and, furthermore, research holes, despite the fact that the fundamental center is to give the headings to future upgrade and advancement around there. This paper gives a deliberate study identified with this calculated model for the cutting edge of exploration, which is talked about in the predefined writing to date. The study is envisioned to be useful in examining the PSO algorithm in detail for the specialist. Likewise, the paper finds the proficiency of numerous quasi-random sequences (QRS) based on initialization approaches by looking at their exhibition analyzed for sixteen notable benchmark test problems.Keywords
Cite This Article
Citations
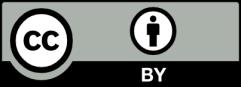
This work is licensed under a Creative Commons Attribution 4.0 International License , which permits unrestricted use, distribution, and reproduction in any medium, provided the original work is properly cited.