Open Access
ARTICLE
Quality Prediction of Wearable Apps in the Google Play Store
1 Department of Computer Science, COMSATS University Islamabad, Attock Campus, 43600, Pakistan
2 Faculty of Computing, The Islamia University of Bahawalpur, Bahawalpur, 63100, Pakistan
3 Department of Computer Science and Software Engineering, International Islamic University, Islamabad, 44000, Pakistan
4 Department of Computer Engineering, College of Computer and Information Technology, Taif University, PO Box 11099, Taif, 21994, Saudi Arabia
5 Faculty of Engineering, Moncton University, NB, E1A3E9, Canada
6 Department of Computer Engineering, Jeju National University, Jejusi, 63243, Korea
7 Department of Information and Communication Engineering, Yeungnam University, Gyeongsan, 38541, Korea
* Corresponding Author: Muhammad Shafiq. Email:
Intelligent Automation & Soft Computing 2022, 32(2), 877-892. https://doi.org/10.32604/iasc.2022.022266
Received 02 August 2021; Accepted 03 September 2021; Issue published 17 November 2021
Abstract
Play Store reviews play an important role in demonstrating that decisions are made from the user’s perspective, and contain a wealth of knowledge that can be used to understand quality issues and help developers build higher-quality mobile applications. Even for very important information, it can ensure the authenticity of user-generated content. In Play Store, wearable applications were recently launched, and are always open and easy to use, and are gradually being welcomed by users. Driven by popularity and self-interest, profit-incentive developers are developing low-quality applications and hiring robots to exaggerate ratings, reviews, or install counts. This is how spam in applications increases. Low-quality applications reduce the user’s quality experience and trust, because after users download an application, they will know the irrelevant and annoying content of the application. As a result, the reputation of the Play Store is damaged. Therefore, we analyzed the review content of different wearable applications and proposed a regression model that has a wide range of recommended features, including sentiment, content similarity, language and time features, to detect wearable applications in the Play Store. We use advanced machine learning techniques to evaluate and verify the quality of the model. Compared with existing models, the performance of our proposed model is very good, with an error rate as low as 0.40 MSE. Therefore, our regression model is most suitable for deep neural network (DNN) training.Keywords
Cite This Article
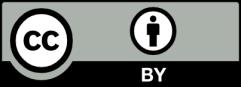
This work is licensed under a Creative Commons Attribution 4.0 International License , which permits unrestricted use, distribution, and reproduction in any medium, provided the original work is properly cited.