Open Access
ARTICLE
Forecasting of Trend-Cycle Time Series Using Hybrid Model Linear Regression
1 Department of Computer Science and Engineering, BMS Institute of Technology and Management, Bangalore, 560064, India
2 Department of Computer Science and Engineering, Acharya Institute of Technology, Bangalore, 560107, India
3 Manuro Tech Research Pvt. Ltd., Bangalore, 560097, India
* Corresponding Author: N. Ashwini. Email:
Intelligent Automation & Soft Computing 2022, 32(2), 893-908. https://doi.org/10.32604/iasc.2022.022231
Received 31 July 2021; Accepted 02 September 2021; Issue published 17 November 2021
Abstract
Forecasting for a time series signal carrying single pattern characteristics can be done properly using function mapping-based principle by a well-designed artificial neural network model. But the performances degraded very much when time series carried the mixture of different patterns characteristics. The level of difficulty increases further when there is a need to predict far time samples. Among several possible mixtures of patterns, the trend-cycle time series is having its importance because of its occurrence in many real-life applications like in electric power generation, fuel consumption and automobile sales. Over the mixed characteristics of patterns, a neural model, suffered heavily in getting generalized learning, in result poor performances appeared over test data. To overcome this issue in this work, a decomposition-based approach has been applied to separate the component patterns of trend and cyclic patterns, and a dedicated model has been developed for predicting the individual data patterns. The linear characteristic of the trend data pattern has been modeled through a linear regression model while the nonlinearity behavior of cyclic pattern has been model by an adaptive radial basis function neural network. The final predicted outcome has been considered as the linear combination of individual model outcomes. The Gaussian function has been considered as the kernel function in the radial basis function neural network because of its wider and efficient applicability in function mapping. The performance of the neural model has been improved very much by providing the adaptive value of spreads and centers of basis function along with weights values. In this paper, two different applications of forecasting in the area of electric power demand by the individual house and month-wise annual power generation have been considered. Based on house characteristics parameters, the power demanded by a house have been considered which carried a moderate complexity of function mapping problem while in another case, total power generation needed to be predicted on the monthly basis for a year from just the previous year observation, which carried the mixed behavior of trend and cyclic pattern. For house power demand forecasting the adaptive kernel-based radial basis function has shown very satisfactory performances and much better against static kernel radial basis function and multilayer perceptron neural network. The integrated approach of neural model and linear regression has shown very efficient outcomes for the mixture pattern while individual neural models were failed to do so. The coefficient of determination (R2) has been applied to estimate the quality of predicted outcomes and comparison.Keywords
Cite This Article
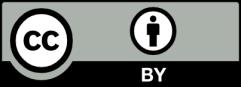
This work is licensed under a Creative Commons Attribution 4.0 International License , which permits unrestricted use, distribution, and reproduction in any medium, provided the original work is properly cited.