Open Access
ARTICLE
Deep Neural Networks for Gun Detection in Public Surveillance
1 Department of Computer Science, Superior University, Lahore, 54000, Pakistan
2 Department of Informatics and Systems, University of Management and Technology, Lahore, 54000, Pakistan
3 Faculty of Computer Science and Information Technology, Superior University, Lahore, 54000, Pakistan
4 Department of Software Engineering, Superior University, Lahore, 54000, Pakistan
* Corresponding Author: Erssa Arif. Email:
Intelligent Automation & Soft Computing 2022, 32(2), 909-922. https://doi.org/10.32604/iasc.2022.021061
Received 21 June 2021; Accepted 26 August 2021; Issue published 17 November 2021
Abstract
The conventional surveillance and control system of Closed-Circuit Television (CCTV) cameras require human resource supervision. Almost all the criminal activities take place using weapons mostly handheld gun, revolver, or pistol. Automatic gun detection is a vital requirement now-a-days. The use of real-time object detection system for the improvement of surveillance is a promising application of Convolutional Neural Networks (CNN). We are concerned about the real-time detection of weapons for the surveillance cameras, so we focused on the implementation and comparison of faster approaches such as Region (R-CNN) and Region Fully Convolutional Networks (R-FCN) with feature extractor Visual Geometry Group (VGG) and ResNet respectively. Training and testing are done on database that consists of local environment images. These images are taken with different type and high- resolution cameras that minimize the idealism. Some metrics also defined to reduce the false positives which are specific to the solution of problem. This research also contributes to the constitution of a hybrid CNN model of both faster-based R-CNN and R-FCN. Both hybrid and existing models experimented to reduce false positive in weapon detection. Result represented in graph with calculation during and after training with confusion matrix and hybrid model results better than other models.Keywords
Cite This Article
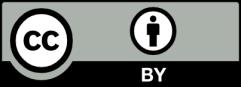