Open Access
ARTICLE
Emotion Recognition with Short-Period Physiological Signals Using Bimodal Sparse Autoencoders
1 School of Electrical Engineering, Korea University, 145, Anam-ro, Seongbuk-gu, Seoul, Korea
2 Department of Software, Sangmyung University, Cheonan, 31066, Korea
3 Department of System Semiconductor Enginnering, Sangmyung University, Cheonan, 31066, Korea
4 Department of Human Intelligence and Robot Engineering, Sangmyung University, Cheonan, 31066, Korea
* Corresponding Author: Tae-Koo Kang. Email:
Intelligent Automation & Soft Computing 2022, 32(2), 657-673. https://doi.org/10.32604/iasc.2022.020849
Received 11 June 2021; Accepted 06 September 2021; Issue published 17 November 2021
Abstract
With the advancement of human-computer interaction and artificial intelligence, emotion recognition has received significant research attention. The most commonly used technique for emotion recognition is EEG, which is directly associated with the central nervous system and contains strong emotional features. However, there are some disadvantages to using EEG signals. They require high dimensionality, diverse and complex processing procedures which make real-time computation difficult. In addition, there are problems in data acquisition and interpretation due to body movement or reduced concentration of the experimenter. In this paper, we used photoplethysmography (PPG) and electromyography (EMG) to record signals. Firstly, we segmented the emotion data into 10-pulses during preprocessing to identify emotions with short period signals. These segmented data were input to the proposed bimodal stacked sparse auto-encoder model. To enhance recognition performance, we adopted a bimodal structure to extract shared PPG and EMG representations. This approach provided more detailed arousal-valence mapping compared with the current high/low binary classification. We created a dataset of PPG and EMG signals, called the emotion dataset dividing into four classes to help understand emotion levels. We achieved high performance of 80.18% and 75.86% for arousal and valence, respectively, despite more class classification. Experimental results validated that the proposed method significantly enhanced emotion recognition performance.Keywords
Cite This Article
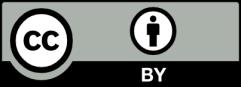
This work is licensed under a Creative Commons Attribution 4.0 International License , which permits unrestricted use, distribution, and reproduction in any medium, provided the original work is properly cited.