Open Access
ARTICLE
MSM: A Method of Multi-Neighborhood Sampling Matching for Entity Alignment
1 School of Artificial Intelligence, Wuxi Vocational College of Science and Technology, Wuxi, 214028, China
2 School of Astronautics, Harbin Institute of Technology, Harbin, 150001, China
3 Department of Mathematics, Harbin Institute of Technology, Weihai, 264209, China
4 School of Computer Science and Technology, Harbin Institute of Technology, Weihai, 264209, China
5 School of Computer Science, University College Dublin, Dublin, Dublin4, Ireland
* Corresponding Author: Xiaofang Li. Email:
Intelligent Automation & Soft Computing 2022, 32(2), 1141-1151. https://doi.org/10.32604/iasc.2022.020218
Received 14 May 2021; Accepted 15 June 2021; Issue published 17 November 2021
Abstract
The heterogeneity of knowledge graphs brings great challenges to entity alignment. In particular, the attributes of network entities in the real world are complex and changeable. The key to solving this problem is to expand the neighborhoods in different ranges and extract the neighborhood information efficiently. Based on this idea, we propose Multi-neighborhood Sampling Matching Network (MSM), a new KG alignment network, aiming at the structural heterogeneity challenge. MSM constructs a multi-neighborhood network representation learning method to learn the KG structure embedding. It then adopts a unique sampling and cosine cross-matching method to solve different sizes of neighborhoods and distinct topological structures in two entities. To choose the right neighbors, we apply a down-sampling process to select the most informative entities towards the central target entity from its one-hop and two-hop neighbors. To verify the effectiveness of matching this neighborhood with any neighborhood in the corresponding node, we give a cosine cross-graph neighborhood matching method and conduct detailed research and analysis on three entity matching datasets, which proves the effectiveness of MSM.Keywords
Cite This Article
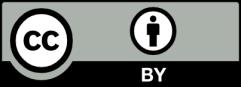