Open Access
ARTICLE
Machine Learning for Modeling and Control of Industrial Clarifier Process
1 Department of Mechatronics, Jyothi Engineering College, Thrissur, 679531, India
2 Department of Computer Science, Dambi Dollo University, Dambi Dollo, 260, Ethiopia
3 Department of Mechanical Engineering, Theni Kammavar Sangam College of Technology, Theni, 625534, India
4 COMBA I+D Research Group of Universidad Santiago de Cali, 760036, Colombia
5 Al-Nahrain Nano renewable Energy Research Center, Al-Nahrain University, Baghdad, 10001, Iraq
* Corresponding Author: C. Karthik. Email:
(This article belongs to the Special Issue: Soft Computing Methods for Intelligent Automation Systems)
Intelligent Automation & Soft Computing 2022, 32(1), 339-359. https://doi.org/10.32604/iasc.2022.021696
Received 11 July 2021; Accepted 17 August 2021; Issue published 26 October 2021
Abstract
In sugar production, model parameter estimation and controller tuning of the nonlinear clarification process are major concerns. Because the sugar industry’s clarification process is difficult and nonlinear, obtaining the exact model using identification methods is critical. For regulating the clarification process and identifying the model parameters, this work presents a state transition algorithm (STA). First, the model parameters for the clarifier are estimated using the normal system identification process. The STA is then utilized to improve the accuracy of the system parameters that have been identified. Metaheuristic algorithms such as Genetic Algorithm (GA), Particle Swarm Optimization (PSO), and State Transition Algorithm are used to evaluate the most accurate model generated by the algorithms. By capturing the principal dynamic features of the process, the clarifier model produced from State Transition Algorithm (STA) acts more like the actual clarifier process. According to the findings, the controllers provided in this paper may be used to achieve greater performance than the standard controller design during the control of any nonlinear procedure, and STA is extremely helpful in modeling a nonlinear process.Keywords
Cite This Article
Citations
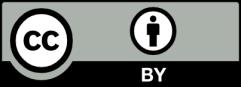
This work is licensed under a Creative Commons Attribution 4.0 International License , which permits unrestricted use, distribution, and reproduction in any medium, provided the original work is properly cited.