Open Access
ARTICLE
Massive IoT Malware Classification Method Using Binary Lifting
1 ISAA Lab., Department of AI Convergence Network, Ajou University, Suwon, 16499, Korea
2 Department of AI Convergence Network, Department of Cyber Security, Ajou University, Suwon, 16499, Korea
* Corresponding Author: Jin Kwak. Email:
Intelligent Automation & Soft Computing 2022, 32(1), 467-481. https://doi.org/10.32604/iasc.2022.021038
Received 20 June 2021; Accepted 23 July 2021; Issue published 26 October 2021
Abstract
Owing to the development of next-generation network and data processing technologies, massive Internet of Things (IoT) devices are becoming hyperconnected. As a result, Linux malware is being created to attack such hyperconnected networks by exploiting security threats in IoT devices. To determine the potential threats of such Linux malware and respond effectively, malware classification through an analysis of the executed code is required; however, a limitation exists in that each heterogeneous architecture must be analyzed separately. However, the binary codes of a heterogeneous architecture can be translated to a high-level intermediate representation (IR) of the same format using binary lifting and malicious behavior information can be identified because the functions and parameters of the assembly code are stored in the IR. Consequently, this study suggests a Linux malware classification method applicable to various architectures by converting Linux assembly codes into an IR using binary lifting and then learning the IR Sequence which reflects malicious behavior pattern using deep learning model for sequence learning.Keywords
Cite This Article
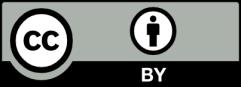
This work is licensed under a Creative Commons Attribution 4.0 International License , which permits unrestricted use, distribution, and reproduction in any medium, provided the original work is properly cited.