Open Access
ARTICLE
Heart Failure Patient Survival Analysis with Multi Kernel Support Vector Machine
1 School of Information Technology & Engineering, Vellore Institute of Technology, Vellore, 632014, India
2 Lord Buddha Education Foundation & Scientific Research Group in Egypt (SRGE), Kathmandu, 44600, Nepal
3 School of Computer Science and Engineering SCE, Taylors University, Slangor, 47500, Malaysia
4 Material Science Research Institute, King Addulaziz City for Science and Technology (KACST), Riyad, 6086, Kingdom of Saudi Arabia
5 General Administration of Research and Development Laboratories, King Abdulaziz City for Science and Technology (KACST), Riyad, 6086, Kingdom of Saudi Arabia
* Corresponding Author: Zahrah A. Almusaylim. Email:
Intelligent Automation & Soft Computing 2022, 32(1), 115-129. https://doi.org/10.32604/iasc.2022.019133
Received 03 April 2021; Accepted 05 May 2021; Issue published 26 October 2021
Abstract
Heart failure (HF) is an intercontinental pandemic influencing in any event 26 million individuals globally and is expanding in commonness. HF healthiness consumptions are extensive and will increment significantly with a maturing populace. As per the World Health Organization (WHO), Cardiovascular diseases (CVDs) are the major reason for all-inclusive death, taking an expected 17.9 million lives per year. CVDs are a class of issues of the heart, blood vessels and include coronary heart sickness, cerebrovascular illness, rheumatic heart malady, and various other conditions. In the medical care industry, a lot of information is as often as possible created. Nonetheless, it is frequently not utilized adequately. The information shows that the produced picture, sound, text, or record has some shrouded designs and their connections. Devices used to remove information from these data sets for clinical determination of illness or different reasons for existing are more uncommon. 4 cases out of 5 CVD dying are due to heart attacks and strokes, 33% of these losses of life happen roughly in peoples under 70 year of age. In the current work, we have tried to predict the survival chances of HF sufferers using methods such as attribute selection (scoring method) & classifiers (machine learning). The scoring methods (SM) used here are the Gini Index, Information Gain, and Gain Ratio. Correlation-based feature selection (CFS) with the best first search (BFS) strategy for best attribute selection (AS). We have used multi-kernel support vector machine (MK-SVM) classifiers such as Linear, Polynomial, radial base function (RBF), Sigmoid. The classification accuracy (CA) we received using SM is as follows: SVM (Linear with 80.3%, Polynomial with 86.6%, RBF with 83.6%, Sigmoid with 82.3%) and by using CFS-BFS method are as follows: SVM (Linear with 79.9%, Polynomial with 83.3%, RBF and Sigmoid with 83.6%).Keywords
Cite This Article
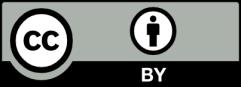
This work is licensed under a Creative Commons Attribution 4.0 International License , which permits unrestricted use, distribution, and reproduction in any medium, provided the original work is properly cited.