Open Access
ARTICLE
IoT and Machine Learning Based Stem Borer Pest Prediction
1 Govt. Post Graduate College Burewala, 61010, Pakistan
2 The Superior University Lahore, 54000, Pakistan
3 The University of Agriculture Faisalabad Sub Campus, Burewala, 61010, Pakistan
* Corresponding Author: Rana Muhammad Saleem. Email:
(This article belongs to the Special Issue: Soft Computing Methods for Intelligent Automation Systems)
Intelligent Automation & Soft Computing 2022, 31(3), 1377-1392. https://doi.org/10.32604/iasc.2022.020680
Received 03 June 2021; Accepted 11 July 2021; Issue published 09 October 2021
Abstract
Global climatic changes have severe impacts on agricultural productivity. Enhanced pest attacks on crops are one of the major impacts on sustainable developments in agriculture to come up with the needs of the ever-increasing human population. Early warning of a pest attack is important for Integrated Pest Management (IPM) activities to be effective. Early warning of pest attacks is also important for judicious use of pesticides for efficient use of resources for minimal impacts on the environment. Sugarcane is the major cash crop and is also severely affected by different types of pests. This study proposed stem borer attack prediction on sugarcane crops by directly sensed environment conditions from the crop field using the Internet of Things (IoT). Data-driven machine learning decisions are made to predict the pest population from directly sensed crop field temperature, humidity, and rainfall conditions. Directly sensed environment conditions and the data-driven decision by the Naïve Bays classification approach help to accurately predict the occurrence of a pest attack above or below the Economic Threshold Level (ETL). The performance of the proposed solution is judged in terms of the performance of the machine learning model and the accuracy of the proposed solution in the prediction of the stem borer attack on the sugarcane crop. The main objective of the proposed solution is to support sustainable developments in agriculture by using the IoT to capture the crop field context and machine learning to make data-driven decisions.Keywords
Cite This Article
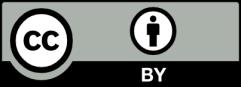