Open Access
ARTICLE
IIoT Framework Based ML Model to Improve Automobile Industry Product
1 Research Scholar, Anna University, Chennai, Tamilnadu, India
2 Department of EEE, SSN College of Engineering, Chennai, Tamilnadu, India
* Corresponding Author: S. Gopalakrishnan. Email:
Intelligent Automation & Soft Computing 2022, 31(3), 1435-1449. https://doi.org/10.32604/iasc.2022.020660
Received 02 June 2021; Accepted 11 July 2021; Issue published 09 October 2021
Abstract
In the automotive industry, multiple predictive maintenance units run behind the scenes in every production process to support significant product development, particularly among Accessories Manufacturers (AMs). As a result, they wish to maintain a positive relationship with vehicle manufacturers by providing 100 percent quality assurances for accessories. This is only achievable if they implement an effective anticipatory strategy that prioritizes quality control before and after product development. To do this, many sensors devices are interconnected in the production area to collect operational data (humanity, viscosity, and force) continuously received from machines and sent to backend computers for control operations and predictive analysis. As a result, there is a vast volume of data that may be processed further to obtain accurate information on equipment processing speed and production efficiency. However, extracting details in the essential format for data-driven decision support for predictive maintenance is problematic. As a result, an effective predictive maintenance approach based on Machine Learning (ML) methods is established. It has an impact on the Hybrid Machine Learning (HML) model, which blends supervised and unsupervised learning. It helps to forecast breakdowns and production line deviations ahead of time, preventing the manufacturing unit from shutting down. The proposed predictive methodology has been tested in terms of earlier anomaly detection, production line accuracy & machinery efficiency and compared with other existing ML based predictive maintenance approaches.Keywords
Cite This Article
Citations
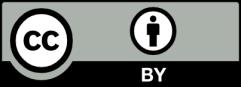
This work is licensed under a Creative Commons Attribution 4.0 International License , which permits unrestricted use, distribution, and reproduction in any medium, provided the original work is properly cited.