Open Access
ARTICLE
Classification Framework for COVID-19 Diagnosis Based on Deep CNN Models
1 Department of Electronics and Electrical Communications, Faculty of Electronic Engineering, Menoufia University, Menouf, 32952, Egypt
2 Department of Information Technology, College of Computer and Information Sciences, Princess Nourah Bint Abdulrahman University, Riyadh, 84428, Saudi Arabia
3 Department of Industrial Electronics and Control Engineering, Faculty of Electronic Engineering, Menoufia University, Menouf, 32952, Egypt
4 Department of Electronics and Communications Engineering, Faculty of Engineering, Zagazig University, Zagazig, 44519, Egypt
* Corresponding Author: Abeer D. Algarni. Email:
Intelligent Automation & Soft Computing 2022, 31(3), 1561-1575. https://doi.org/10.32604/iasc.2022.020386
Received 22 May 2021; Accepted 11 July 2021; Issue published 09 October 2021
Abstract
Automated diagnosis based on medical images is a very promising trend in modern healthcare services. For the task of automated diagnosis, there should be flexibility to deal with an enormous amount of data represented in the form of medical images. In addition, efficient algorithms that could be adapted according to the nature of images should be used. The importance of automated medical diagnosis has been maximized with the evolution of COVID-19 pandemic. COVID-19 first appeared in China, Wuhan, and then it has exploded in the whole world with a very bad impact on our daily life. The third wave of COVID-19 in the third world is really a disaster in current days, especially with the emergence of the delta variant of COVID-19 that is widespread. Required inspections should be carried out to monitor the COVID-19 spread in daily life and allow primary diagnosis of suspected cases, and long-term clinical laboratory monitoring. Healthcare professionals or radiologists can exploit AI (Artificial Intelligence) tools to quickly and reliably identify the cases of COVID-19. This paper introduces a DCNN (Deep Convolutional Neural Network) framework for chest X-ray and CT image classification based on TL (Transfer Learning). The objective is to perform multi-class and binary classification of the images in order to determine pneumonia and COVID-19 case. The TL is feasible, when using a small dataset by transferring knowledge from natural image classification to medical image classification. Two types of TL are used. The first type is fine-tuning of the DenseNet121, Densenet169, DenseNet201, ResNet50, ResNet152, VGG16, and VGG19 models. The second type is deep tuning of the LeNet-5, AlexNet, Inception naïve v1, and VGG16 models. Extensive tests have been carried out on datasets of chest X-ray and CT images with different training/testing ratios of 80%:20%, 70%:30%, and 60%:40%. Experimental results on 9,270 chest X-ray ray and 2,762 chest CT images acquired from different institutions show that the TL is effective with an average accuracy of 98.49%.Keywords
Cite This Article
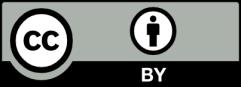
This work is licensed under a Creative Commons Attribution 4.0 International License , which permits unrestricted use, distribution, and reproduction in any medium, provided the original work is properly cited.