Open Access
ARTICLE
Enhancing Detection of Malicious URLs Using Boosting and Lexical Features
Princess Sumaya University for Technology, Amman, 11941, Jordan
* Corresponding Author: Mohammad Atrees. Email:
Intelligent Automation & Soft Computing 2022, 31(3), 1405-1422. https://doi.org/10.32604/iasc.2022.020229
Received 15 May 2021; Accepted 17 June 2021; Issue published 09 October 2021
Abstract
A malicious URL is a link that is created to spread spams, phishing, malware, ransomware, spyware, etc. A user may download malware that can adversely affect the computer by clicking on an infected URL, or might be convinced to provide confidential information to a fraudulent website causing serious losses. These threats must be identified and handled in a decent time and in an effective way. Detection is traditionally done through the blacklist usage method, which relies on keyword matching with previously known malicious domain names stored in a repository. This method is fast and easy to implement, with the advantage of having low false-positive rates regarding previously recognized malicious URLs. However, this method cannot recognize newly created malicious URLs. To solve this problem, many machine-learning models have been used. In this paper, we introduce an effective machine learning approach that uses an ensemble learner algorithm called AdaBoost (Adaptive Boosting), combined with different algorithms that enhance detection. For datasets filtration, we used CfsSubsetEval technique, which is an algorithm that searches for a subset of features that work well together. Datasets were collected from the UNB repository; divided into four categories: spam, phishing, malware, and defacement URLs; combined with benign URLs, dataset content is based on lexical features. The experimental results indicate that the proposed approach was successful in enhancing the detection accuracy of malicious URLs with less false-positive rates for all experimental algorithms.Keywords
Cite This Article
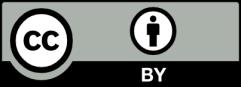
This work is licensed under a Creative Commons Attribution 4.0 International License , which permits unrestricted use, distribution, and reproduction in any medium, provided the original work is properly cited.