Open Access
ARTICLE
An Energy Aware Algorithm for Edge Task Offloading
1 Beijing University of Posts and Telecommunications, Beijing, 100876, China
2 Communication Operation Center State Grid Henan Electric Power Company, Information & Telecommunication Company, Zheng Zhou, 450052, China
3 Fibrlink Communications Co., Ltd, Beijing, 102200, China
4 Global Energy Interconnection Research Institute Co., Ltd, Beijing, 102200, China
5 Université Paul Sabatier-Toulouse 35, Toulouse, 31000, France
6 China Electronics Standardization Institute, Beijing, 100007, China
* Corresponding Author: Meng Chen. Email:
Intelligent Automation & Soft Computing 2022, 31(3), 1641-1654. https://doi.org/10.32604/iasc.2022.018881
Received 24 March 2021; Accepted 29 April 2021; Issue published 09 October 2021
Abstract
To solve the problem of energy consumption optimization of edge servers in the process of edge task unloading, we propose a task unloading algorithm based on reinforcement learning in this paper. The algorithm observes and analyzes the current environment state, selects the deployment location of edge tasks according to current states, and realizes the edge task unloading oriented to energy consumption optimization. To achieve the above goals, we first construct a network energy consumption model including servers’ energy consumption and link transmission energy consumption, which improves the accuracy of network energy consumption evaluation. Because of the complexity and variability of the edge environment, this paper designs a task unloading algorithm based on Proximal Policy Optimization (PPO), besides we use Dijkstra to determine the connection path between edge servers where adjacent tasks are deployed. Finally, lots of simulation experiments verify the effectiveness of the proposed method in the process of task unloading. Compared with contrast algorithms, the average energy saving of the proposed algorithm can reach 22.69%.Keywords
Cite This Article
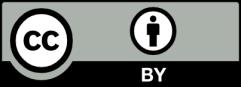
This work is licensed under a Creative Commons Attribution 4.0 International License , which permits unrestricted use, distribution, and reproduction in any medium, provided the original work is properly cited.