Open Access
ARTICLE
PCN2: Parallel CNN to Diagnose COVID-19 from Radiographs and Metadata
1 Department of Computer Engineering, College of Computer and Information Systems, Umm Al-Qura University, Makkah, 21955, Saudi Arabia
2 Department of Computer Engineering, College of Computers and Information Technology, Taif University, Taif, 21944, Saudi Arabia
* Corresponding Author: Mohammed Baz. Email:
Intelligent Automation & Soft Computing 2022, 31(2), 1051-1069. https://doi.org/10.32604/iasc.2022.020304
Received 19 May 2021; Accepted 01 July 2021; Issue published 22 September 2021
Abstract
COVID-19 constitutes one of the devastating pandemics plaguing humanity throughout the centuries; within about 18 months since its appearing, the cumulative confirmed cases hit 173 million, whereas the death toll approaches 3.72 million. Although several vaccines became available for the public worldwide, the speed with which COVID-19 is spread, and its different mutant strains hinder stopping its outbreak. This, in turn, prompting the desperate need for devising fast, cheap and accurate tools via which the disease can be diagnosed in its early stage. Reverse Transcription Polymerase Chain Reaction (RTPCR) test is the mainstay tool used to detect the COVID-19 symptoms. However, due to the high false-negative rate of this test, physicians usually use chest radiographs as an adjunct or alternative tool. Although radiographs screening is wide-available, low-cost, and its results are timely, relying on radiologists to interpret them manually stands against using radiographs as a diagnostic tool. Motivated by the need to speed up the radiographic diagnosis of COVID-19 and to improve its reliability, this paper proposes a novel deep-learning framework dubbed Parallel Deep Neural Networks for COVID-19 Diagnosis (PCN2). PCN2 treats the radiographs and their metadata simultaneously by running two CNNs in parallel. Firstly: a 2-dimensional CNN (2DCNN) to capture the spatial information from the radiographs due to its super competency in this domain. Secondly, a 1-dimensional CNN (1DCNN) to extract the medical knowledge presented in the metadata. By this integration, PCN2 can make perfect classifications even for those cases in which the infection signs in radiographs are unclear due to being the disease in early-stage, confounded by other markers or overlapped by other diseases. Extensive assessments of PCN2 carried out using several datasets demonstrate average diagnostic accuracy of 99.9 and 0.99 F1-score.Keywords
Cite This Article
Citations
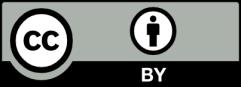
This work is licensed under a Creative Commons Attribution 4.0 International License , which permits unrestricted use, distribution, and reproduction in any medium, provided the original work is properly cited.