Open Access
ARTICLE
Severity Grade Recognition for Nasal Cavity Tumours Using Décor CNN
Department of Computer Science and Engineering, School of Computing, SRM Institute of Science and Technology, Chengalpattu, Tamil Nadu, 603203, India
* Corresponding Author: Prabhakaran Mathialagan. Email:
Intelligent Automation & Soft Computing 2022, 31(2), 929-946. https://doi.org/10.32604/iasc.2022.020163
Received 12 May 2021; Accepted 18 June 2021; Issue published 22 September 2021
Abstract
Nasal cavity and paranasal sinus tumours that occur in the respiratory tract are the most life-threatening disease in the world. The human respiratory tract has many sites which has different mucosal lining like frontal, parred, sphenoid and ethmoid sinuses. Nasal cavity tumours can occur at any different mucosal linings and chances of prognosis possibility from one nasal cavity site to another site is very high. The paranasal sinus tumours can metastases to oral cavity and digestive tracts may lead to excessive survival complications. Grading the respiratory tract tumours with dysplasia cases are more challenging using manual pathological procedures. Manual microscopic biopsy tissue analysis is time consuming and chances of occurring interobserver variability problems are very high. To avoid nasal cavity tumour grade misclassification a State-Of-The-Art Automated Décor CNN model is proposed. The automated nasal cavity tumour grading classification model is designed to troubleshoot the stain artifact present in the biopsy tissue samples. Major nasal cavity sites are analysed to find the abnormal epithelium. The automated morphological characteristic findings with décor CNN model in biopsy images are promising. The model achieves the classification accuracy of 98% with less training, testing and validation errors compared to other transfer learning convolution neural network models.Keywords
Cite This Article
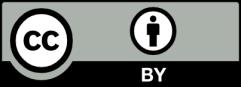
This work is licensed under a Creative Commons Attribution 4.0 International License , which permits unrestricted use, distribution, and reproduction in any medium, provided the original work is properly cited.