Open Access
ARTICLE
Intelligent Audio Signal Processing for Detecting Rainforest Species Using Deep Learning
1 Department of CSE, Chandigarh University, Punjab, India
2 Department of Computer Science, College of Computer Sciences and Information Technology, King Faisal University, Al-Ahsa, 31982, Saudi Arabia
* Corresponding Author: Shakeel Ahmed. Email:
Intelligent Automation & Soft Computing 2022, 31(2), 693-706. https://doi.org/10.32604/iasc.2022.019811
Received 26 April 2021; Accepted 14 June 2021; Issue published 22 September 2021
Abstract
Hearing a species in a tropical rainforest is much easier than seeing them. If someone is in the forest, he might not be able to look around and see every type of bird and frog that are there but they can be heard. A forest ranger might know what to do in these situations and he/she might be an expert in recognizing the different type of insects and dangerous species that are out there in the forest but if a common person travels to a rain forest for an adventure, he might not even know how to recognize these species, let alone taking suitable action against them. In this work, a model is built that can take audio signal as input, perform intelligent signal processing for extracting features and patterns, and output which type of species is present in the audio signal. The model works end to end and can work on raw input and a pipeline is also created to perform all the preprocessing steps on the raw input. In this work, different types of neural network architectures based on Long Short Term Memory (LSTM) and Convolution Neural Network (CNN) are tested. Both are showing reliable performance, CNN shows an accuracy of 95.62% and Log Loss of 0.21 while LSTM shows an accuracy of 93.12% and Log Loss of 0.17. Based on these results, it is shown that CNN performs better than LSTM in terms of accuracy while LSTM performs better than CNN in terms of Log Loss. Further, both of these models are combined to achieve high accuracy and low Log Loss. A combination of both LSTM and CNN shows an accuracy of 97.12% and a Log Loss of 0.16.Keywords
Cite This Article
R. Kumar, M. Gupta, S. Ahmed, A. Alhumam and T. Aggarwal, "Intelligent audio signal processing for detecting rainforest species using deep learning," Intelligent Automation & Soft Computing, vol. 31, no.2, pp. 693–706, 2022. https://doi.org/10.32604/iasc.2022.019811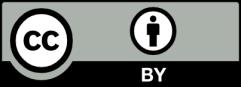