Open Access
ARTICLE
Multi-Model Detection of Lung Cancer Using Unsupervised Diffusion Classification Algorithm
1 Department of Computer Science and Engineering, KPR Institute of Engineering and Technology, Coimbatore, 641407, Tamilnadu, India
2 Department of Computer Science and Engineering, St. Joseph’s Institute of Technology, Chennai, 600119, Tamilnadu, India
3 Department of Medical Equipment Technology, College of Applied Medical Sciences, Majmaah University, Al Majmaah, 11952, Saudi Arabia
4 Department of EEE, SRM Institute of Science and Technology, Chennai, 603203, India
* Corresponding Author: N. Jayanthi. Email:
Intelligent Automation & Soft Computing 2022, 31(2), 1317-1329. https://doi.org/10.32604/iasc.2022.018974
Received 28 March 2021; Accepted 25 June 2021; Issue published 22 September 2021
Abstract
Lung cancer is a curable disease if detected early, and its mortality rate decreases with forwarding treatment measures. At first, an easy and accurate way to detect is by using image processing techniques on the cancer-affected images captured from the patients. This paper proposes a novel lung cancer detection method. Firstly, an adaptive median filter algorithm (AMF) is applied to preprocess those images for improving the quality of the affected area. Then, a supervised image edge detection algorithm (SIED) is presented to segment those images. Then, feature extraction is employed to extract the mean, standard deviation, energy, contrast, etc., of the cancer-affected area. Finally, an unsupervised diffusion classification (UDC) algorithm is explored to narrow down the affected areas. The proposed lung cancer detection method is implemented on two datasets obtained from standard hospital real-time values. The experiment results achieved superior performance in the detection of lung cancer, which demonstrates that our new model can contribute to the early detection of lung cancer.Keywords
Cite This Article
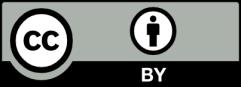
This work is licensed under a Creative Commons Attribution 4.0 International License , which permits unrestricted use, distribution, and reproduction in any medium, provided the original work is properly cited.