Open Access
ARTICLE
Selecting Dominant Features for the Prediction of Early-Stage Chronic Kidney Disease
Rajalakshmi Engineering College, Chennai, Tamilnadu, India
* Corresponding Author: Vinothini Arumugam. Email:
Intelligent Automation & Soft Computing 2022, 31(2), 947-959. https://doi.org/10.32604/iasc.2022.018654
Received 16 March 2021; Accepted 17 June 2021; Issue published 22 September 2021
Abstract
Nowadays, Chronic Kidney Disease (CKD) is one of the vigorous public health diseases. Hence, early detection of the disease may reduce the severity of its consequences. Besides, medical databases of any disease diagnosis may be collected from the blood test, urine test, and patient history. Nevertheless, medical information retrieved from various sources is diverse. Therefore, it is unadaptable to evaluate numerical and nominal features using the same feature selection algorithm, which may lead to fallacious analysis. Applying machine learning techniques over the medical database is a common way to help feature identification for CKD prediction. In this paper, a novel Mixed Data Feature Selection (MDFS) model is proposed to select and filter preeminent features from the medical dataset for earlier CKD prediction, where CKD clinical data with 12 numerical and 12 nominal features are fed to the MDFS model. For each feature in the mixed dataset, the model applies feature selection methods according to the data type of the feature. Point Biserial correlation and a Chi-square filter are applied to filter the numerical features and nominal features, respectively. Meanwhile, an SVM algorithm is employed to evaluate and select the best feature subset. In our experimental results, the proposed MDFS model performs superior to existing works in terms of accuracy and the number of reduced features. The identified feature subset is also demonstrated to preserve its original properties without discretization during feature selection.Keywords
Cite This Article
Citations
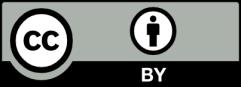
This work is licensed under a Creative Commons Attribution 4.0 International License , which permits unrestricted use, distribution, and reproduction in any medium, provided the original work is properly cited.